In an industry that prides itself on innovation and progress, the observability sector stands as an anomaly – a field seemingly trapped in amber, where genuine evolution has been replaced by an endless cycle of marketing-driven rebranding. Despite decades of supposed advancement, the fundamental approaches to understanding system behavior remain stubbornly unchanged, masked only by increasingly elaborate terminology and ever-growing data collection.
The COBOL of Engineering
If observability were a programming language, it’d be COBOL – not because of its age but because of its stubborn resistance to evolution. While other sectors of technology have seen genuine paradigm shifts – from the rise of cloud computing to the transformation of database technologies – observability remains locked in patterns established decades ago. The core practices – logging, metrics, and distributed tracing – haven’t fundamentally changed; they’ve merely been repackaged with new buzzwords and increasingly complex query interfaces.
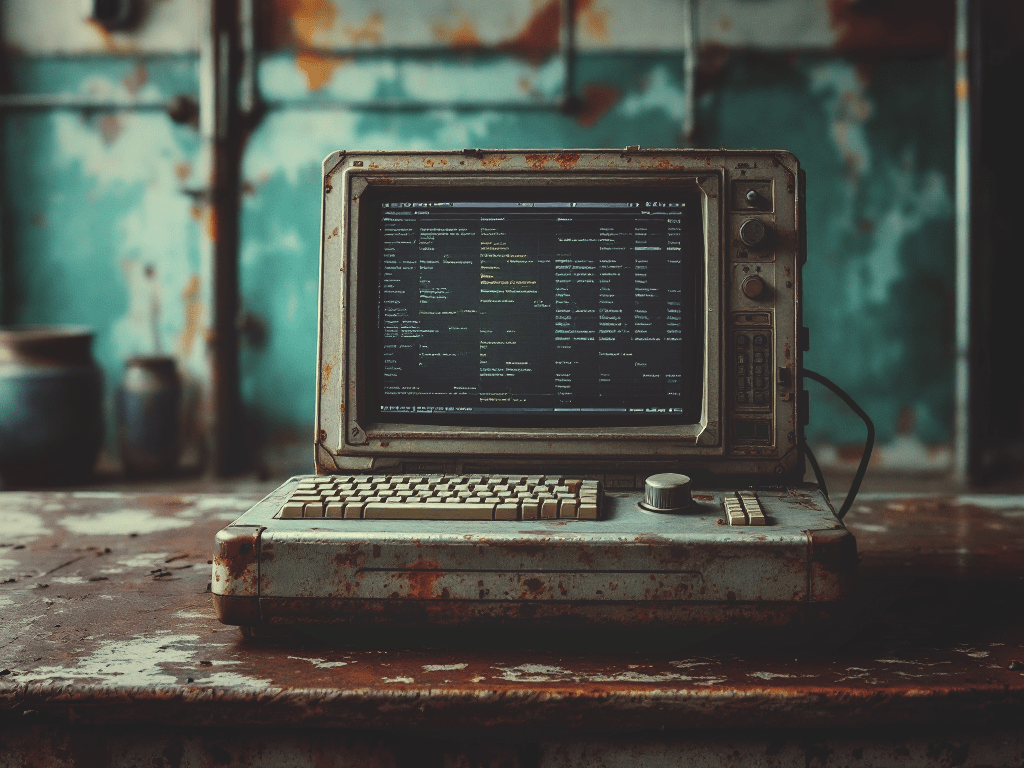
The Walking Dead
The current state of observability is akin to a technology industry zombie apocalypse as depicted in The Walking Dead, where practitioners and vendors persistently repeat the same motions, episode after episode, season after season.
The industry seems incapable of finding a solution through logical reasoning, instead doubling down on approaches that have consistently failed to provide a comprehensive understanding of system behavior.
Just as survivors in zombie narratives often fortify themselves in isolated strongholds, engineering teams barricade themselves behind walls of logging tools, metrics dashboards, and trace visualizations.
These defensive measures provide an illusion of safety and control, but they fragment our understanding rather than unify it. Each tool becomes its shelter, disconnected from the broader landscape of system behavior.
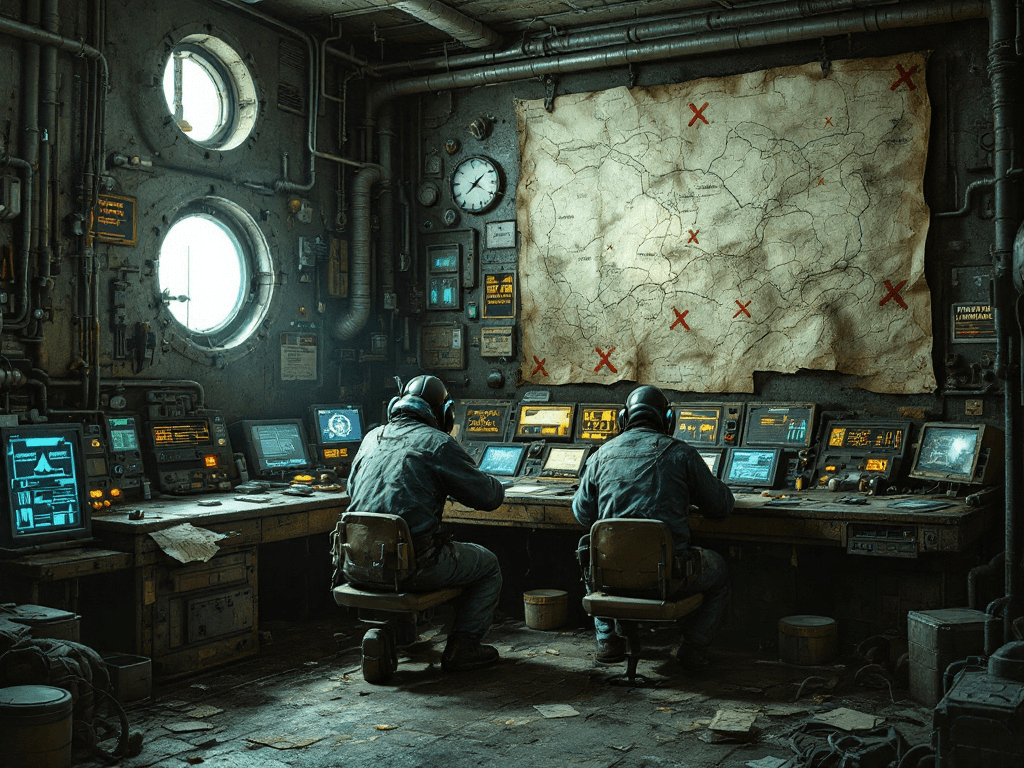
The zombification of observability manifests in the mindless consumption of system data without genuine comprehension. Like the undead who endlessly shamble forward without a purpose, our monitoring systems collect vast amounts of telemetry that lurches through our dashboards, never quite capturing the essence of what makes our systems truly alive or dead. We gather metrics, logs, and traces with an insatiable hunger, but this feast of data often leaves us paradoxically malnourished in terms of actual insight.
The path forward requires us to break free from this cycle of mindless repetition and tool proliferation.
We need to evolve our approach from mere survival to the revival of what observability should be: a means of deeply understanding our systems’ behavior, not just monitoring their vital signs. This means developing new mental models, embracing holistic approaches to system comprehension, and perhaps most importantly, acknowledging that our current shambling approach to observability needs more than just another weapon upgrade – it needs a cure.
Lipstick on a Pig
The observability sector’s approach to innovation resembles putting new lipstick on the same pig. Each year brings new vendors promising revolutionary approaches, but beneath the glossy marketing and buzzword-laden presentations lie the same basic techniques from decades past. The basic approach to system understanding remains unchanged, merely decorated with increasingly ornate marketing cosmetics. This endless cycle of rebranding without fundamental innovation keeps the industry stuck in patterns established before many of today’s challenges even existed.
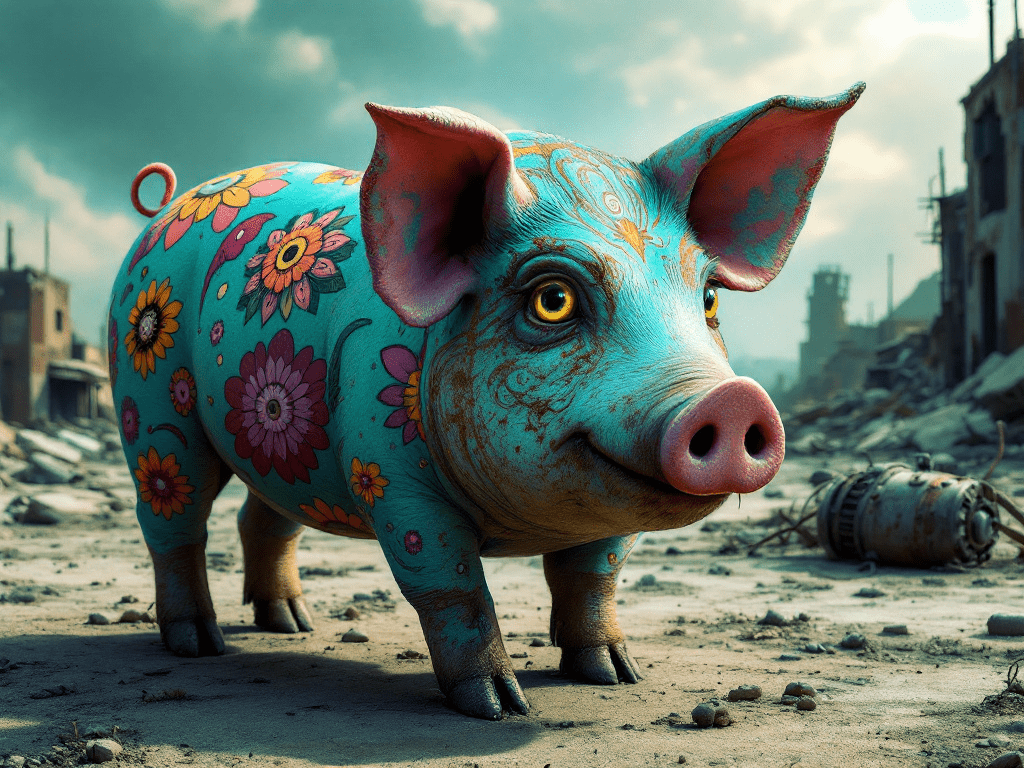
What the industry needs isn’t another coat of paint – it needs a fundamental reimagining of how we approach system understanding in an era where traditional assumptions about application architecture no longer hold true.
The “Unknown Unknowns” Fallacy
Perhaps no concept better illustrates the sector’s dysfunction than the popular narrative around unknown unknowns. This marketing-driven philosophy has led organizations down a path of endless data collection and storage, promising that somewhere in the haystack lies a needle that’ll reveal critical insights. This approach has fostered widespread practices of hoarding excessive data just in case, while organizations struggle with spiraling storage and compute costs.
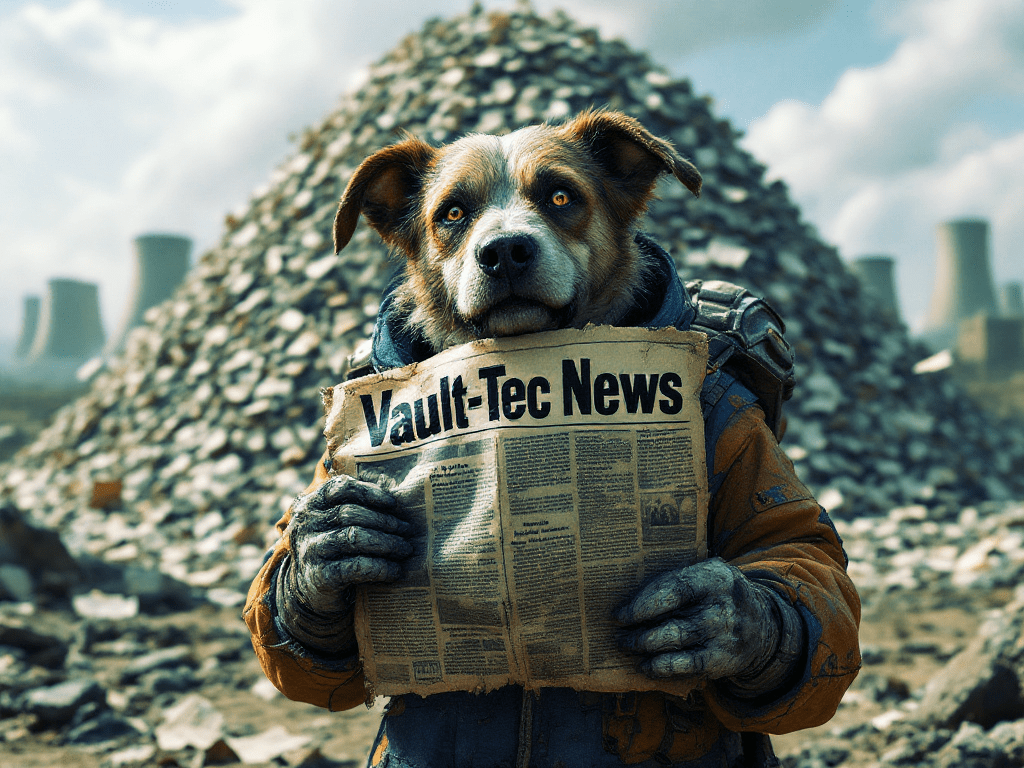
The overwhelming amount of information has decreased operational visibility due to noise, and teams have lost focus on maintaining real-time situational awareness. The result is a form of operational blindness where teams spend more time hunting through historical data than understanding current system dynamics.
Consider how this parallels the evolution of photography in the smartphone era. Just as we now take hundreds of photos without truly looking at what we’re capturing, organizations have adopted a capture-everything mindset that paradoxically makes them less observant of what’s happening in their systems. They’re so busy photographing the moment that they’re not experiencing it. This prioritization of data collection over immediate action has engendered a debilitating organizational distraction. Teams flit between disparate historical analyses, neglecting the pressing need for decisive action and problem-solving in the present; their focus is akin to endlessly browsing a photo album rather than navigating their immediate surroundings. The present, the crucial arena for decision-making, is relegated to an inconsequential afterthought, overshadowed by exhaustive historical data analysis.
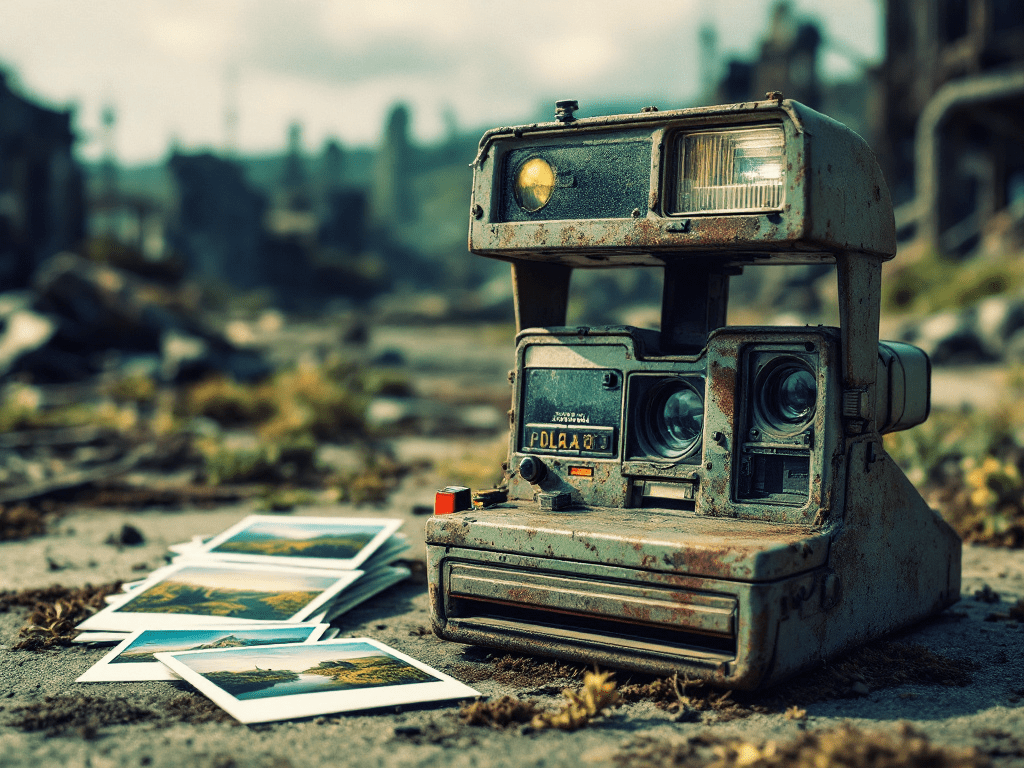
The False Promise of “Semantic” Observability
The latest iteration is called semantic observability, which mistakenly equates adding metadata fields to telemetry data with achieving true semantic understanding. The misunderstanding around semantic observability mirrors a deeper pattern we often see in technology: the belief that quantity can be a substitute for quality in understanding.
This is a fundamental flaw, as genuine semantic understanding necessitates comprehending the relationships, context, and inherent meaning within the data. Adding more attributes to telemetry data without understanding these aspects is akin to believing that simply having more playing cards makes one a better poker player; it doesn’t improve the actual skill or insight. Effective semantic observability requires a deeper integration of contextual information and a sophisticated analysis of the relationships between different data points to extract meaningful insights. This means moving beyond simple tagging and instead building systems capable of interpreting the significance of the data.
The focus should be on creating systems that can understand the meaning of the data, not just its raw form.
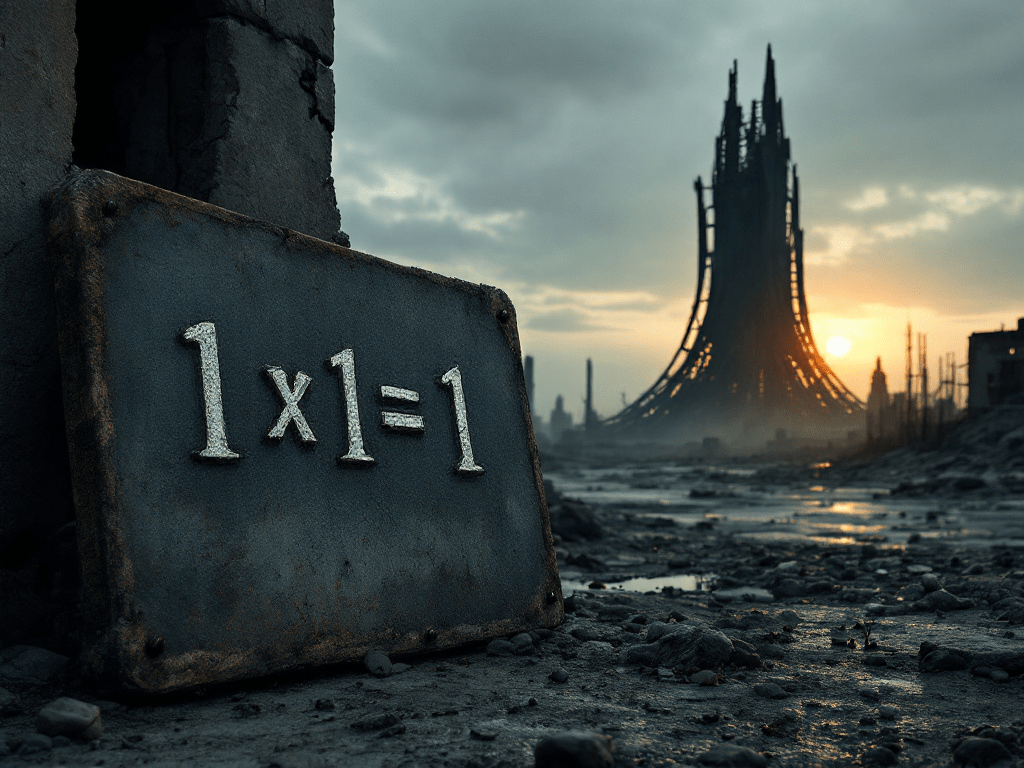
Progress hinges not on data accumulation but on cultivating sophisticated analytical capabilities to decipher data’s true meaning. This necessitates abandoning the more is better fallacy and prioritizing the development of systems capable of holistic, contextual data analysis, akin to an expert poker player’s comprehensive game strategy, recognizing intricate patterns arising from complex interactions.
The Missing Piece: Situational Awareness
Vendor solutions consistently lack a crucial element: situational awareness. This means a real-time grasp of how a system is behaving and any developing issues that operators need to address. Instead, vendors overwhelmingly focus on capabilities that only become useful after a problem has occurred, such as post-incident diagnostics, and ad-hoc queries. This significant gap between what vendors offer and what operations teams need is a major problem.
While vendors promote historical analysis, operations teams urgently require real-time insights into system dynamics to effectively manage their systems and prevent problems before they escalate. This real-time understanding is essential for proactive problem-solving and efficient system management.
This persistent gap between vendor offerings and operational requirements reveals a deeper truth about system management: the most valuable moments for intervention often occur in the subtle precursors to problems, not in their aftermath. Just as a skilled sailor can read subtle changes in wind and waves to adjust their course before a storm hits, operators need tools that help them develop this same level of environmental awareness for their systems.
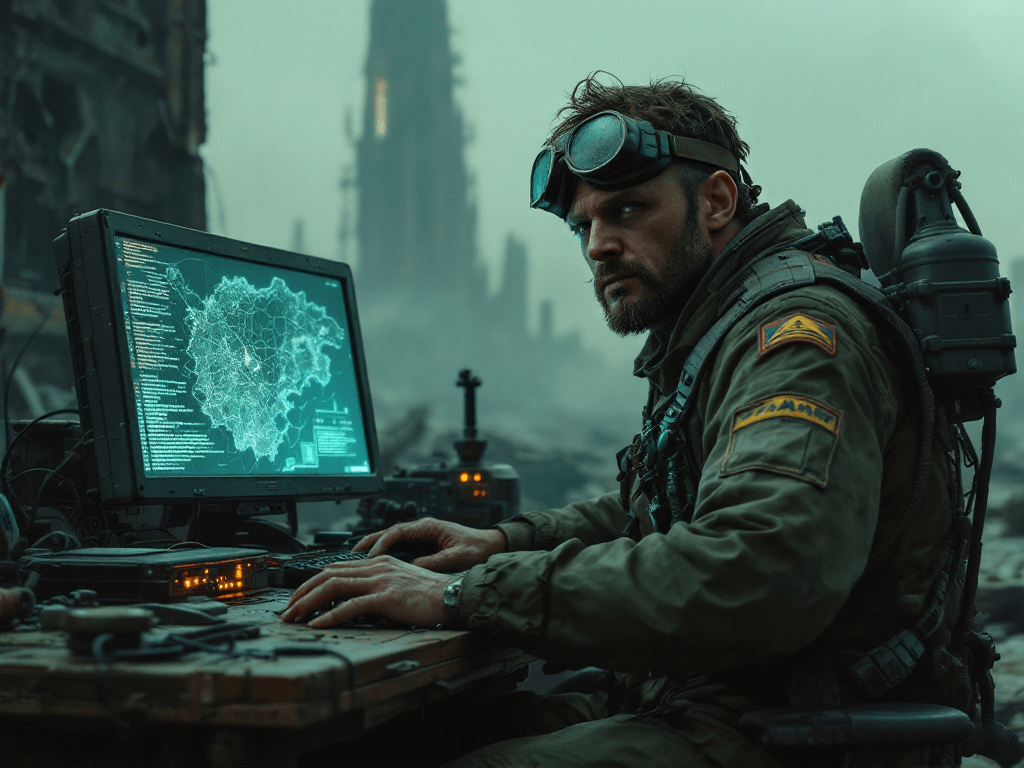
The Self-Perpetuating Cycle
This dysfunction is self-perpetuating. Vendors benefit from keeping organizations focused on collecting more data and building more complex query capabilities rather than developing better approaches to understanding system behavior. The unknown-unknowns narrative creates a perfect justification for endless data collection and storage, while the promise that the next query will reveal crucial insights keeps teams invested in increasingly complex analysis tools.
This dynamic mirrors the phenomenon of security theater in aviation, where visible but ineffective measures create an illusion of safety. In the realm of system observability, we might call this data theater – an elaborate performance where the continuous accumulation of logs and metrics provides a comforting sense of control without necessarily improving our actual understanding.
The market of vendors providing observability tools has grown significantly due to the widespread concern about the complexities of systems. This growth mirrors a historical arms race: just as medieval castles developed increasingly advanced defenses only to spur the creation of even more powerful siege weapons, the constant addition of new data collection and analysis tools to understand complex systems ironically contributes to increased complexity.
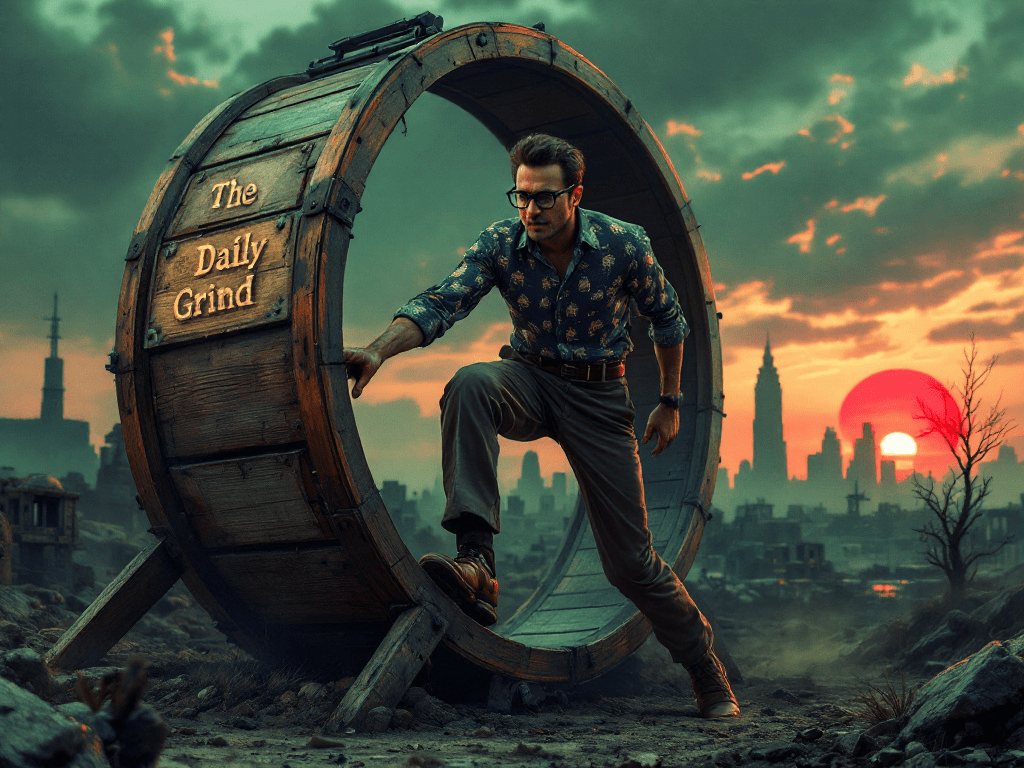
The accretion of observability layers, each with its complexities, paradoxically hinders rather than facilitates comprehension of system behavior. This proliferation of tools generates a data deluge that overwhelms even experienced engineers, obscuring critical issues and impeding actionable intelligence, thereby perpetuating a cycle of escalating system management complexity.
The inherent danger of this pattern lies in its exploitation of our cognitive biases. The sunk cost fallacy traps teams in their current data infrastructure, and confirmation bias reinforces the perceived value of infrequent, successful complex queries, masking the ongoing maintenance burden.
Breaking the Cycle
Progress necessitates a transformative change in our approach to observability. We must move beyond the current focus on accumulating vast amounts of data and designing complex query interfaces. Instead, we need to adopt a comprehensive strategy that prioritizes real-time comprehension of system dynamics and behavioral patterns.
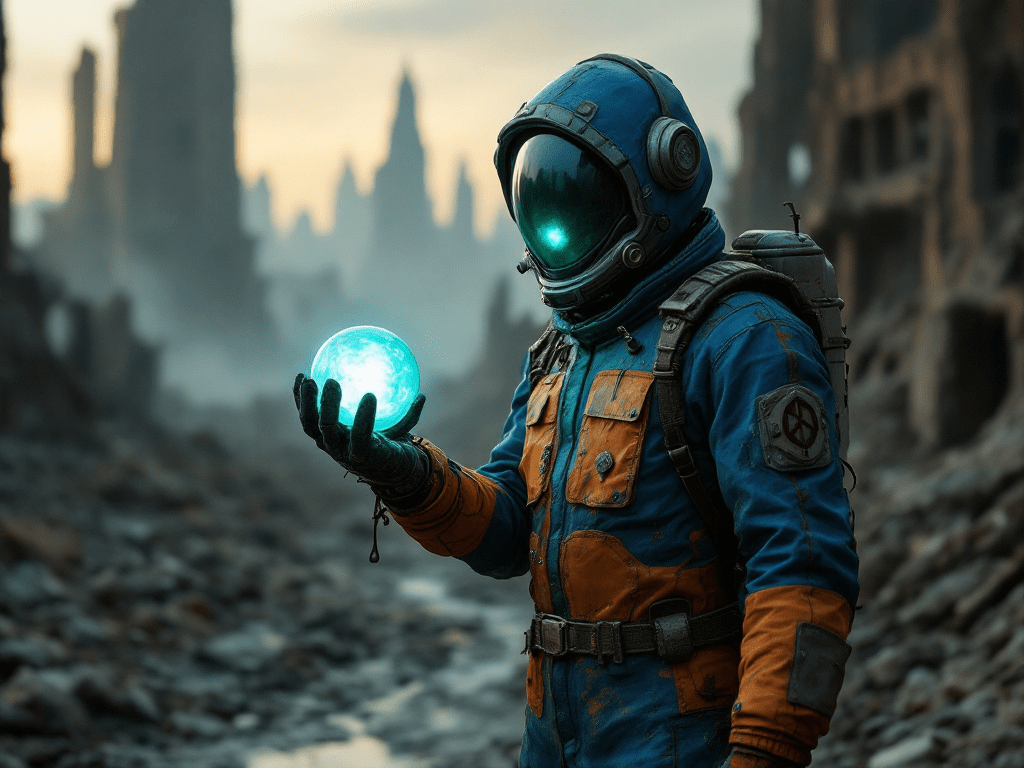
This involves designing frameworks that view systems not as isolated data points but as interconnected, constantly evolving entities. The goal is to build instruments that offer a true understanding of the current state of our systems, revealing their behavior and the interactions between their various components. We need to be able to understand the complex interplay of different parts of the system and how they influence each other in real-time. This allows for proactive identification of issues and improved decision-making.
This transformation isn’t just about better tools – it’s about fundamentally changing how we think about and interact with our systems. We need to cultivate a more holistic, systems-thinking approach that recognizes the dynamic, interconnected nature of modern technology ecosystems. Only then can we move beyond the limitations of our current observability practices and develop the deep understanding needed to build and maintain truly resilient systems.
Conclusion
The fundamental irony in modern observability mirrors the ancient Greek myth of Sisyphus, eternally pushing his boulder up the hill. Just as Sisyphus was condemned to endless repetition, the observability sector keeps implementing variations of the same monitoring patterns established in the early days of distributed systems. We’re essentially building increasingly elaborate ways to watch the boulder roll back down, rather than questioning why we’re pushing it.
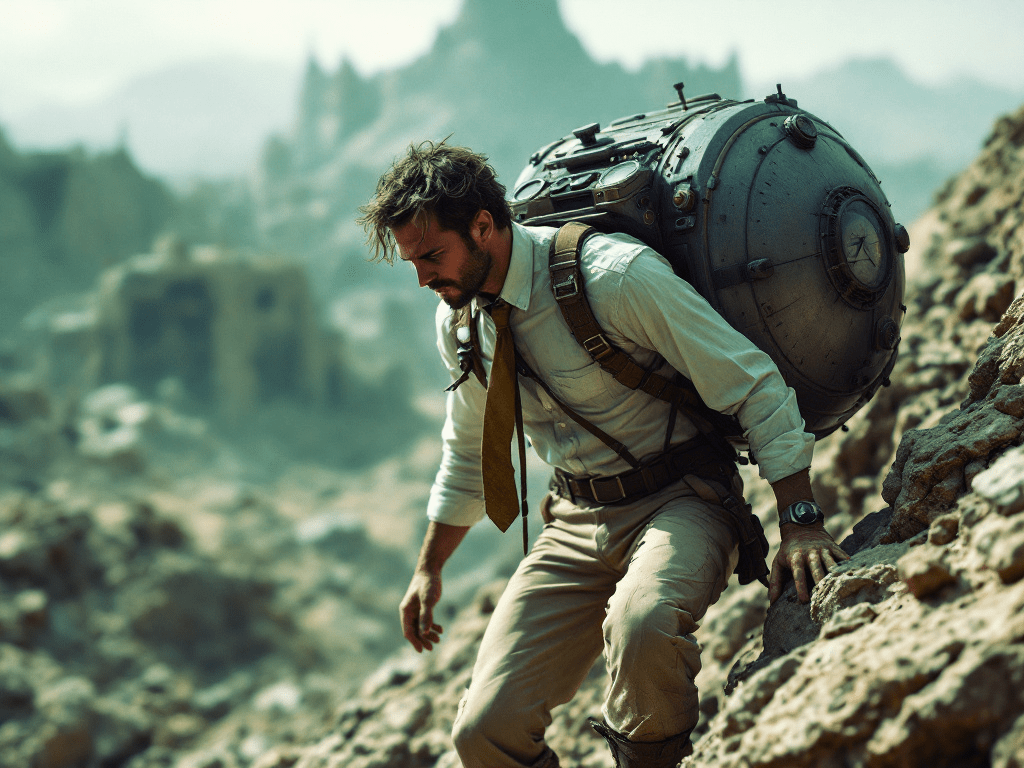
The observability sector is perhaps the most dysfunctional sector in modern technology. It is stuck in patterns established decades ago while merely applying new layers of marketing gloss to old approaches. Breaking free from this cycle requires more than new tools or more data collection—it requires a fundamental rethinking of how we approach understanding system behavior. Until then, we remain trapped in our own technological Groundhog Day, doomed to repeat the same patterns while hoping for different results.