Draft Version: 0.3
In an era where AI is rapidly transforming our digital landscape, how can we ensure that human-AI collaboration reaches its full potential? The answer lies in a paradigm shift towards task-centricity.
Imagine a world where every interaction with an information system is purposeful, where data doesn’t just inform but drives action, and where humans and machines work in perfect harmony. This is the promise of task-centric systems. By integrating four powerful concepts – Promise Theory, Speech Act Theory, Joint Action, and Situation Awareness – task-centricity redefines how we approach service interfaces and user interactions. It’s not just about processing information anymore; it’s about getting things (jobs) done.
The shift to task-centricity represents more than just a technological advancement—it’s a fundamental reimagining of how humans and AI can work together. By focusing on tasks as the primary unit of interaction and discourse, we can build a bridge to close the gap between humans and machines.
In this article, we’ll explore:
– The limitations of traditional data-centric systems
– How task-centricity transforms user experiences
– The role of Promise Theory in fostering autonomous cooperation
– How Speech Act Theory turns words into actions
– The power of Joint Action in coordinating human-AI efforts
Future updates to include:
– How naturally effective Observability is built into a task-centricity system
– How Situation Awareness can be realized in information systems
– The pragmatics of user interface and interaction design in this new paradigm
Tasks: A New Interface Paradigm
Step into a room pulsating with screens, each one flooding with an unending flow of data. You can sense the precious insights hidden within, but how do you unlock them? How do you transform this whirlpool of statistics into a catalyst for real-world change? This is the perplexing puzzle that confronts countless users when dealing with antiquated information systems. In the days of yore, our digital interfaces adhered to a straightforward principle: lay bare the data and leave it to the user to find purpose within. Yet in the swift currents of the modern era, one must ponder if this approach still serves us well.
Task-centricity flips the script. Instead of asking, “What data can we show?”, it asks, “What goal is the user trying to accomplish?” This shift in perspective transforms how we design and interact with digital systems.
By treating each interaction as a task-driven speech act, we unlock the power of promises for coordination, negotiation, and action. This radical shift not only streamlines the process of assigning, accepting, and completing tasks, but it also nurtures autonomous and cooperative behavior.
Creating interfaces based on a clear task model can improve the effectiveness, intuitiveness, and user focus of systems. This approach helps guide users towards specific tasks and presents data in a way that’s relevant to those tasks. As a result, the system becomes more practical, reduces cognitive burden, and promotes confident decision-making. In information systems and decision support systems, a task-centered approach has several benefits compared to the traditional data-centric model. It ensures that users not only access data but can also take effective actions based on it. While data is still essential, placing action or task at the heart of UI/UX design transforms the way users interact with complex systems, making them more dynamic, purposeful, and aligned with the user’s real-world goals.
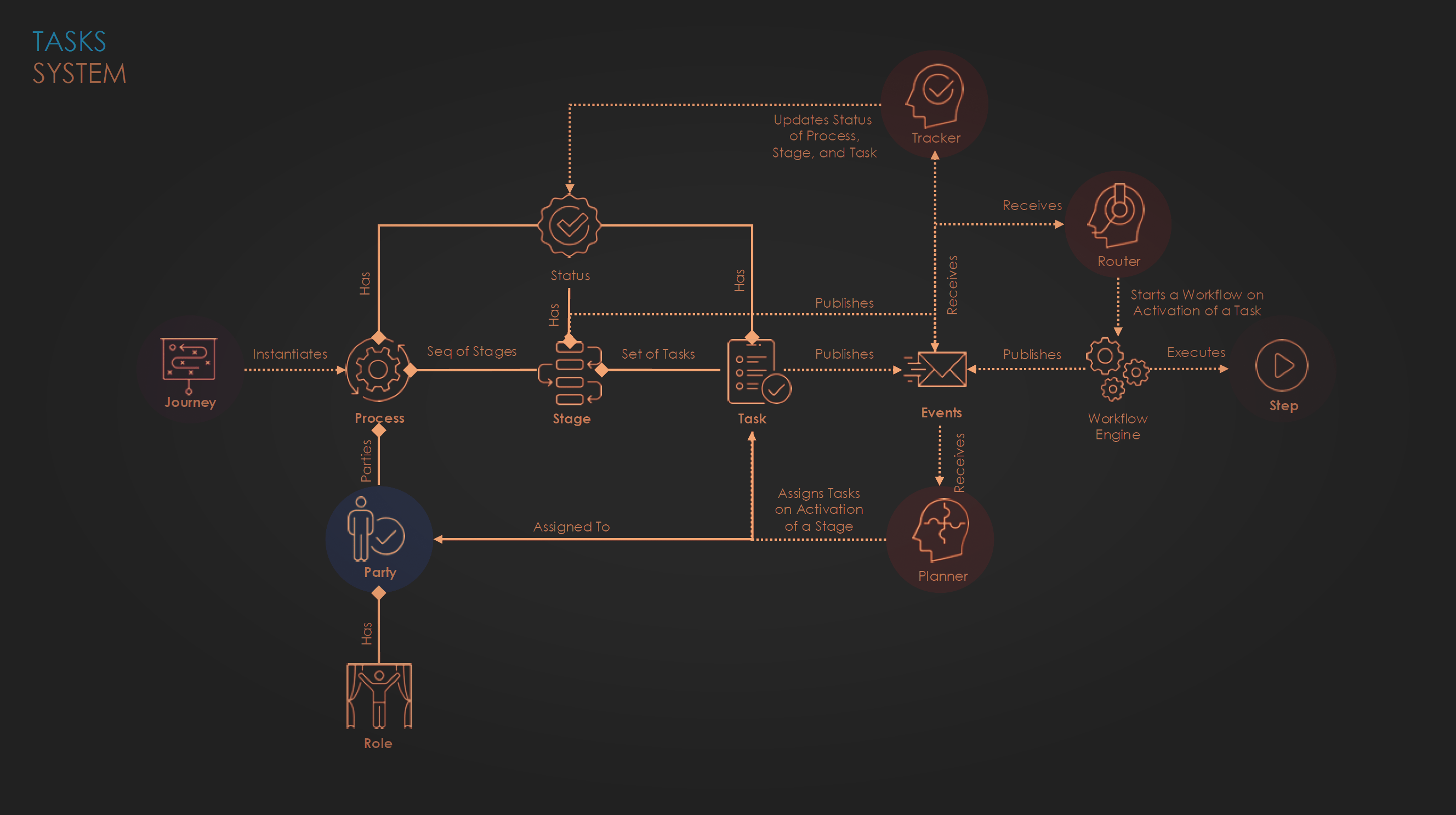
In the above straw-man model, a Journey is a Process type, named and defined in terms of the domain. A Process is an instance of a Journey started by a Party and involving multiple other invited parties. A Process consists of a sequence of Stages. A Stage (or Phase) is a labeled grouping of Tasks. Only one Stage can be active within a Process at a time. A Task is a labeled grouping of Steps, which can be assigned to a Party attached to the Process. A Step is an Action to be executed, typically mapped to a Service endpoint.
At any time a User can have one or more Process instances active. For example, in the case of retail banking, a macro-level Process could represent the journey of the User as a Customer of the bank. A meso-level Process could each represent an individual Product subscription. And finally, a micro-level Process could represent the onboarding process for one particular Product. Even with such a basic model, there’s enough flexibility to allow tailoring to any domain or service offering at various levels of granularity.
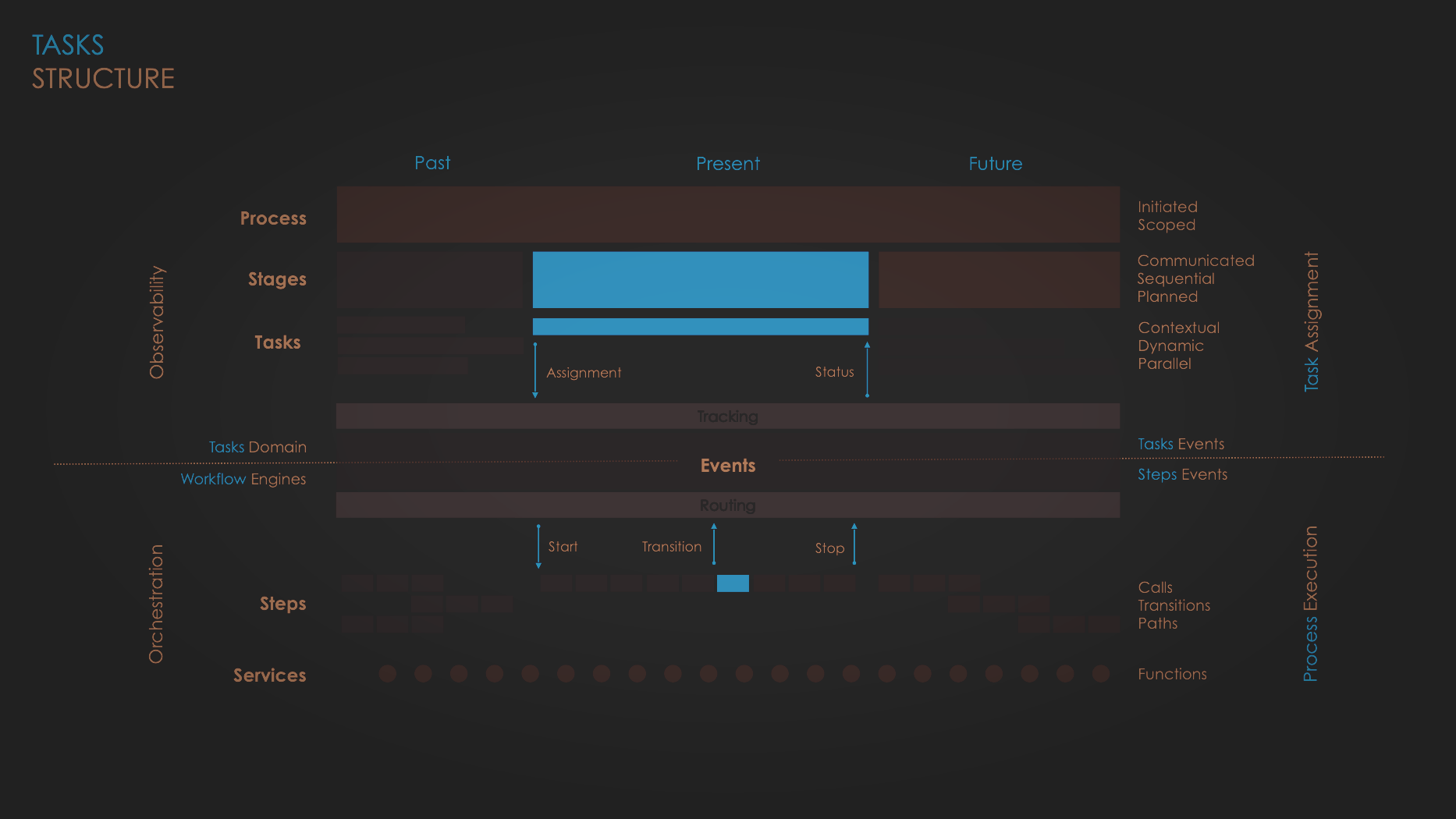
There’s immense power and productivity to be gained in the ease at which storyboards and customer journeys can be mapped, and the implicit structuring of interactions it encourages—where a user conceptualizes primarily in terms of stages, and tasks, while steps and actions update task progress.
Making tasks more visible in the user interface, akin to exposing structural elements in “industrial” architecture, facilitates improved AI-human interaction. This approach promotes functional transparency, enabling users to comprehend and control an AI’s role within a workflow. Consequently, collaboration is enhanced, and user agency is strengthened. This transformation leads to a more integrated and intuitive relationship between humans and AI, which aligns well with the emerging field of explainable AI (XAI), which endeavors to render AI systems more interpretable and comprehensible to humans.
Task-centricity highlights the importance of a dynamic and active system where tasks are treated as ongoing processes rather than mere UI elements. This approach enhances transparency and empowers users by providing insight into the inner workings of manual (humans) and automated (machines) systems.
Instead of presenting static options, tasks are presented as active processes with varying states, and users can have multiple tasks in progress simultaneously. This approach fosters a higher level of user comprehension and control over complex processes, thereby bridging the gap between user actions and the intricate steps involved in digital systems.
Tasks: A Banking Example
The task-centric approach is beneficial for digital financial applications. Users interact with these apps occasionally and with specific goals in mind. Banking systems are complex with many features, but tasks hide this complexity. They only show relevant options, making the app feel simpler. A task-centric approach can also personalize the experience by highlighting recently performed or in-progress tasks.
Nowadays, interactions can occur through various channels. To ensure consistency across different devices like desktop, mobile, and tablet, it’s better to focus on tasks rather than adapting complex navigation and menu structures to different screen sizes. Tasks greatly facilitate the ability for users to seamlessly transition between devices while performing a task. By suspending the task on one device, users can easily resume it on another device without losing any of their progress.
Adopting a task-centric approach provides a holistic and consistent method for managing intricate, multi-stage processes across different systems, which is highly relevant in today’s banking and financial systems. By implementing this universally, we establish a standardized interface for a multitude of activities. Whether it involves a straightforward fund transfer or a complicated loan application, users interact with a familiar framework. By displaying tasks advancing through various stages, we effectively manage user expectations regarding completion times. This can help alleviate user anxiety and reduce the number of inquiries regarding the status of their requests.
Banks commonly use multiple backend systems. This task model offers a unified frontend that can integrate information from various sources, enabling the presentation of a cohesive view to the user. As financial regulations continue to evolve, additional steps or checks may be necessary in processes. Fortunately, this model allows for the seamless integration of new stages without disrupting the user experience. When issues arise, they can be represented as specific task states, facilitating clear communication regarding the problem and the steps being taken to resolve it. As financial products become more intricate, this model effectively scales to represent complex, multi-step processes.
Task-centricity significantly enhances customer support by providing support staff with the ability to view the same task perspective as customers, enabling more efficient and accurate assistance. Implementing this model more explicitly in user interfaces would undeniably yield a higher degree of consistency across various banking processes. It transforms the often opaque world of banking operations into a transparent and comprehensible series of tasks and statuses. This not only enhances the user experience but also aligns the frontend more closely with the actual backend processes, potentially simplifying system architecture and improving overall system reliability and maintainability.
Let’s now dig deeper into the underlying thinking and theory of this audacious and innovative vision.
Tasks: Redefining Digital Interaction
In task-centricity, interactions between humans, machines, and software interfaces are focused on tasks rather than data. This shift in design and function has significant implications for user experience and system functionality. Tasks become the central unit of interaction, with data presented in the context of task-related actions such as assignment, allocation, status, and execution. This new interaction model has profound implications for both how users engage with the system and how the system functions.
Tasks: The Ultimate Productivity Anchor
With a task-centric model, the flow of information and interaction is fundamentally organized around tasks. Each task would act as the anchor for all related data. A user would interact with a system to receive, assign, or reallocate tasks. Data about the task—such as requirements, objectives, and resources—would be shown only when necessary to perform the task.
Tasks: From Data to Action
We must move beyond passive data consumption and make joint action a core aspect of designs. By doing so, we pave the way for interfaces that are not only intelligent and intuitive but also driven by action. This is of the utmost importance as we integrate AI agents more extensively into our systems and workflows. It’s time for us to completely reassess our systems, allowing agentic technologies to flourish and establishing a solid foundation for seamless and productive human-machine partnerships.
Actions: The Ultimate Catalysts
Action fuels communication, coordination, and cooperation. Whether it’s between humans or machines, action is at the core of these interactions. Action is fundamental because it turns potential into reality—words into conversation plans into results, and collaboration into successful outcomes.
Action is how communication, coordination, and cooperation become functional. It serves as the validation that the exchange of ideas or the organization of efforts has succeeded—action provides the essential link between intent and result, making it the foundation of meaningful interaction.
Actions: Communication’s True Engine
When it comes to communication, the main idea is all about sharing information to achieve a response or establish understanding. But it’s not just about words! Actions play a crucial role in determining whether communication is truly successful. This applies not only to human interactions but also to interactions with machines. It’s action that gives communication a clear purpose and structure, making abstract ideas come alive and producing tangible results. Action is the primary means by which messages are sent, received, and interpreted, forming the backbone of any communicative process.
Actions: Speaking Louder Than Words
The essence of coordination lies in aligning efforts between multiple parties to achieve a shared goal. It’s through taking action that humans effectively coordinate, be it through explicit methods such as scheduling and task assignment, or more subtle ways that happen in real-time collaboration.
Within coordination, an outcome is only truly realized when all parties successfully execute their actions. It’s akin to a well-orchestrated performance, where each player must play their part with precision and harmony for the symphony to emerge. Timeliness and accuracy are paramount in coordination, for they lay the foundation of a synchronized workflow that paves the way to success.
Actions: A Symphony of Coordination
Successful collaboration amongst parties is comparable to bees working together to achieve a shared goal. The secret to effective cooperation is the combination of collective efforts towards a common goal. When humans and machines collaborate, with machines enhancing human abilities, the potential for success is limitless. In this partnership, action is what converts cooperation from a mere concept into a productive reality. Without action, cooperation is fruitless, lacking the required productivity to yield outcomes.
Language: Where Words Meet Actions
The role of language in motivating and directing actions is crucial. It’s a powerful tool for issuing commands, making requests, and giving instructions to influence events in the physical world. This aligns with the theory of speech acts, which explores how language is used to achieve specific goals. The theory suggests that utterances can have effects beyond their literal meaning. For example, when someone says “Can you please pass the salt?”, they’re making a request. This theory highlights the performative nature of language and its influence on our interactions and behaviors.
Language: Shaping Action & Reality
In addition to giving commands, language is used to convey the progress of actions—if they’re finished, still happening, or require attention. It helps coordinate actions, plan future steps, and adjust ongoing tasks, thereby strengthening the link between communication and action.
Communication involves more than just sharing information; it involves using words to actively engage with the world. When someone says, “I promise to help you tomorrow,” they aren’t just providing information but making a commitment to take action in the future. Even in situations where physical action isn’t necessary, our use of language functions as actions within social and psychological contexts.
Language: Embodied Action
The idea of embodied cognition, which posits that cognitive processes are inextricably linked to our bodily interactions with the world, reinforces the notion that language is intimately tied to action rather than mere abstract thought. From this perspective, grasping language entails mentally reenacting the described actions, implying that action is not only the result of linguistic exchange but also an essential element in our understanding and expression of language.
Tasks & Actions: Keys to Human-Machine Synergy
Actions serve as the fundamental elements of behavior, whereas tasks represent the structured intentions behind those actions. The distinction between them can be compared to someone diving into a river without any plan versus someone deliberately navigating through the waters. Actions are tangible and can be observed, while tasks involve the management of goals, responsibilities, and results.
Comprehending the concepts of action and task is vital for comprehending human behavior and interaction between humans and machines. The effective assignment, coordination, and supervision of individual actions rely on task management systems, ensuring seamless collaboration between humans and machines like a well-functioning mechanism.
Act & Affect: The Force of Action
Philosophically, action is a more basic and atomic concept than a task. Actions can be simple or complex and are often influenced by decisions or stimuli. They involve actively interacting with the environment, either physically or mentally, to achieve a specific goal. By taking action, we demonstrate our ability to effect change in the world around us. In essence, an action is the realization of an intent in the moment, reflecting agency—the capacity to act and make things happen. Actions are discrete singular events — the smallest unit of behavior that contributes meaningfully towards achieving goals. The focus in action is on immediacy—what’s happening right now—and its success or failure is generally evaluated in terms of the correct execution at the moment. Intelligence is action appropriate to the situation and context.
Tasks: Orchestrating Actions, Achieving Goals
A task is more than just a single action. It’s a structured concept that involves a series of actions with a specific goal. It includes organization, intent, and a desired outcome. Tasks are made up of connected steps that work together towards a bigger purpose. They provide a framework for coordinating actions over time. Achieving a goal through tasks requires intentional effort and collaboration. They’re operational steps that can often be completed independently with minimal context.
A task is more about management and planning. Tasks break down goals into actionable steps, involving assigning roles, setting deadlines, and tracking status. They can be assigned and reassigned as needed to different parties involved in a process. Tasks ensure multiple actions are aligned over time. Tasks are evaluated based on whether they achieve the desired outcome and how efficiently they’re carried out, factoring in the coordination of different actions. The status of a task changes, whereas an action is either executed or not, though the outcome, the required effect, from the action can vary due to other factors.
Steps & Actions: The Harmonious Duo Navigating Tasks
A step serves as the vital foundation of a task, infusing it with structure and vitality. It transcends mere units and embodies a potent concept that divides the task into manageable segments. Each step orchestrates a precise sequence of one or more actions harmonizing to achieve a specific goal.
Steps delineate the necessary actions, while actions function as the means of execution. Steps as guiding beacons that navigate us through the intricate labyrinth of a task, akin to a captain mapping out a course. Actions, in turn, serve as the propelling force that drives us forward, tangible occurrences and operations that transform these guiding beacons into concrete realities. However, steps and actions are more than mere collaborators; they possess an inseparable connection. They dance in perfect synchronization, intricately intertwined to maintain a delicate equilibrium. Steps offer the framework, the contextual backdrop within which actions flourish. At the same time, actions breathe life into each step, serving as the pulse that elevates the abstract and passive into a tangible and dynamic outcome.
Steps possess the potential for repetition and refinement, which in turn facilitate the emergence of new, necessary actions to be executed. While steps may initially appear abstract and passive, they always imply and rely on action. If a step doesn’t lead to any action, it ceases to be a meaningful part of a task. It may be a placeholder or instruction without realization. In this sense, a step without action is like a plan without execution—it might exist as an idea but has no real-world effect.
Today: Data Consumption First, Action Second
In traditional information systems and decision support systems (DSS), the primary focus is often on presenting data to users. Once data is presented, action or task execution becomes secondary, often left to the user to interpret and then act upon based on their conclusions. In this design paradigm, the interface is often passive, presenting data and assuming that users will determine what actions to take next. This passive consumption of data, where action comes later or as a secondary thought, has certain limitations. It creates an experience where users may feel overwhelmed with information or unsure about what specific steps to take next.
Tomorrow: Empowering Users
When a system is designed around the concept of a task, it becomes more aligned with the goals of human users. Information systems are often used by individuals who not only seek to consume data but also have specific goals in mind, such as making a decision, completing a task, or solving a problem.
By structuring the interface in a way that promotes task completion rather than passive data consumption, the system can effectively guide users toward clear and actionable outcomes. Instead of overwhelming users with excessive information, the system can present relevant data directly linked to the task at hand, assisting users in navigating complexity more intuitively and purposefully. For instance, a financial decision support system (DSS) designed around “optimizing investment portfolio” would provide actionable insights, such as suggesting adjustments to increase returns, rather than solely presenting historical stock prices. This approach streamlines the decision-making process by seamlessly integrating relevant data with actionable steps.
Tomorrow: Reducing Cognitive Load
Having interfaces that are overloaded with data can overwhelm users and cause cognitive overload. This happens when users are presented with an excessive amount of information and are left to determine which is the most important. However, if the interface is designed with a task-oriented approach, it can act as a filter, displaying only the necessary information for the specific task at hand. A task-driven interface provides users with a structured pathway and indicates which actions need to be performed, when they should be done, and what data they should be based on. By filtering and prioritizing data based on its relevance to the user’s task, the system becomes more efficient and eliminates unnecessary distractions.
Tomorrow: Streamling Steering
In systems where the primary focus isn’t on taking action, users often find it challenging to convert the available data into actionable decisions. This leaves them feeling unsure about what steps they should take next. On the other hand, in an action-oriented interface, the emphasis is on facilitating prompt decision-making driven by the context. The interface presents the data in a way that suggests specific actions that the user can take. This approach enhances user confidence by offering clear and practical suggestions, thereby minimizing the user’s need to independently analyze the data and devise their plan of action.
Tomorrow: Automation and AI Collaboration
A task-centered design naturally opens up opportunities for automation and collaboration between human and machine actions. By designing a system that focuses on tasks, the system can propose or automate certain tasks directly, saving users time and reducing decision fatigue. Tasks can be suggested or initiated based on real-time data, allowing the system to act more like an intelligent assistant.
Task-Centricity: The System
The task-centric system is a revolutionary approach that prioritizes task execution based on a data-driven approach. This paradigm shift challenges conventional information systems by placing tasks at the core of operations. Instead of solely analyzing and interpreting data, the task-centric system integrates it within the context of task assignment, allocation, status, and execution. The implementation of this approach promises a multitude of benefits, including improved user experience and enhanced system functionality.
One of the key advantages of the task-centric system is its ability to streamline task allocation. By providing a comprehensive overview of task ownership, current progress, and task interdependencies, both users and systems can effortlessly manage and prioritize tasks. Moreover, collaboration becomes more structured and efficient, owing to the system’s ability to automatically notify users of any changes in task assignments, status, or the need for input from others. For instance, as soon as one user completes a task, it seamlessly transitions to the next responsible person or system for the following task.
Overall, the task-centric system offers a game-changing approach that optimizes task management and improves collaboration. Its integration of data within task execution empowers users with a comprehensive perspective of their responsibilities, enabling them to make well-informed decisions and maximize productivity. By leveraging the task-centric system, organizations can unlock significant gains in efficiency and effectiveness. In the end, this model changes how users use information systems. It helps them move from just reading information to doing tasks, making decisions, and taking action.
Task-Centricity: The Journey
In a task-oriented system such as this, where tasks are organized into stages, processes like a customer onboarding journey aren’t simply a linear list of actions. Instead, they represent a dynamic journey that evolves. This journey, when depicted as a process, can be divided into stages, each with its own specific goals. These stages can then be further subdivided into tasks, steps, and ultimately actions. Within this framework, tasks aren’t standalone steps, but rather essential elements of a larger process that unfolds gradually and is interconnected with other tasks, both prior and proceeding. This hierarchical structure creates opportunities for intelligent agents to operate at various levels, ranging from executing individual tasks to engaging in strategic planning.
Task-Centricity: Multi-Agents
The system described here, with many agents—intelligent, AI-driven, and dynamic—operating within a task-based framework, is a sophisticated model that introduces intelligent planning, task allocation, and execution across different stages of a process, such as customer onboarding. In this environment, agents are not only executing tasks but also planning, monitoring, and adapting the system’s workflow in real-time. This kind of multi-agent task system introduces several interesting dynamics and possibilities.
Task-Centricity: Intelligent Planning
Intelligent planning agents, humans or machines, oversee and organize tasks based on the context and objectives of the current stage or the larger journey. Agents can analyze data generated by completed and ongoing tasks to identify new needs, gaps, or opportunities for improvement. Agents can also look to upcoming stages and pre-scheduling tasks that need to be activated later. Planners are more strategic and proactive, ensuring that the entire process moves smoothly by preemptively addressing potential bottlenecks or issues, whether through AI insights or human expertise.
Task-Centricity: Task Mastery
In task management, active agents play a crucial role in executing and supervising tasks in real-time. These agents not only monitor and keep track of the progress of each task and its corresponding actions but also make the necessary adjustments to ensure timely completion. Additionally, they actively engage in communication with planners, providing essential feedback on the status of the tasks. This valuable feedback enables planners to swiftly adapt and respond to any changing conditions or circumstances.
Active agents not only collect and evaluate data from completed tasks to provide insights into the system’s performance, but they can also learn and enhance their capabilities over time. This allows them to become more proficient in task assignments, offering valuable suggestions for improvements. To illustrate, artificial intelligence monitoring agents can identify patterns where a particular set of customers consistently abandon the onboarding process at a specific stage. In such cases, these agents can propose modifications to enhance the customer journey and boost retention rates. By consistently offering feedback, these monitoring agents play a crucial role in fine-tuning execution and improving efficiency.
Task-Centricity: Supercharging Efficiency
A multi-agent task system with AI and human collaboration creates significant opportunities for improved efficiency and decision-making, especially in dynamic, complex processes like customer journeys, project management, or industrial operations. By allowing multiple agents to handle different aspects of task management (planning, execution, monitoring), the system becomes highly adaptable.
Tasks can be redefined, reassigned, or delayed dynamically based on real-time feedback, creating a self-regulating ecosystem. This can lead to faster task completion, as AI agents can automate many of the repetitive or time-consuming decisions (such as reassigning tasks) that’d otherwise slow down progress. Here, tasks are no longer just isolated actions; they become dynamic, interdependent units of a larger, adaptive process. Intelligent agents, both human and machine, can not only execute tasks but also plan, monitor, and schedule future tasks, optimizing workflows in real-time.
Task-Centricity: A Stepping Stone to Agentic-AI
Integrating tasks as the central component in user interfaces and interactions is a fundamental step in advancing Agentic-AI systems. This task-based approach establishes a well-suited framework for incorporating intelligent agents who can actively engage in, execute, plan, and optimize these tasks. By adopting this framework, the development of agentic agents is facilitated. The proposed framework provides a solid structure for agents to understand, acquire knowledge from, and improve upon, enabling them to engage with tasks logically and sequentially.
Agents are no longer solely executors, but also coordinators and advisors, enhancing both the efficiency and intelligence of the system. By framing interactions in terms of tasks, valuable datasets are generated for agents to learn from. Tasks create natural feedback loops, generating new data upon completion, which allows agents to continuously refine task processes, ultimately enhancing the overall efficiency and user experience of the system. This approach fosters a collaborative interface, where humans and AI agents work together to achieve goals, leveraging their respective strengths.
Promise Theory: Decentralized Agent Cooperation
Mark Burgess’s Promise Theory provides a powerful conceptual framework for thinking about collaboration and coordination between autonomous agents—both human and machine—in a distributed system. It emphasizes decentralization, autonomy, and voluntary cooperation between agents, where each agent makes promises about its behavior and capabilities, rather than being centrally controlled or commanded. Promise Theory provides a theoretical foundation for how agents can interact and cooperate within a system where tasks are the core unit of interaction. In this task-centric paradigm, agents commit to tasks. Each task is essentially a promise that an agent makes to complete a particular action within a certain context. This mirrors Promise Theory, where each agent makes voluntary promises about what it’ll do, without necessarily having external enforcement.
Promise Theory: Intent, Not Obligation
According to Promise Theory, a promise is a declaration of intent rather than an obligation. This distinction is essential when dealing with systems that involve agents, whether they be AI, human, or a combination of both. These agents must be able to negotiate and modify their commitments based on their capabilities, current situation, and external factors. In the context of tasks, an AI agent might make a promise to complete a specific task such as data processing or providing a recommendation. On the other hand, a human agent could promise to review the output of the AI or make a decision based on it. Each promise is specific to the agent and the task it’s responsible for. In an environment where agents work together on tasks, the voluntary nature of promises ensures that the system is adaptable and resilient, enabling it to respond to changing conditions without strict centralized control.
Promise Theory: Collaboration via Promises
In Promise Theory, agents establish promises to one another based on their capabilities, current status, and contextual factors. Similarly, within your task-oriented system, both AI and human agents engage in negotiations to allocate tasks based on real-time data, current workload, and expected outcomes. For instance, an AI agent may commit to monitoring the progress of a task and involving a human agent only if any delays or errors arise. On the other hand, human agents may pledge to handle more complex tasks while entrusting routine tasks to the AI. This form of collaboration among agents closely resembles Promise Theory, as agents willingly offer commitments considering their capacities and circumstances, without any coercion. This approach guarantees that tasks are assigned to the most suitable agents, thereby enhancing overall efficiency and effectiveness.
Promise Theory: Contextual & Conditional
Promise Theory highlights the importance of promises being made within a specific context. Within the task-centric paradigm, tasks or promises are integrated into processes, stages, and contexts. Both AI and human agents operate within a larger system of tasks and interactions when making promises. For instance, an AI agent might commit to automating a specific section of the onboarding process, but only if the customer has provided the necessary data. Consequently, promises aren’t isolated events and may require renegotiation or may not be applicable beyond that specific context. The contextual nature of tasks ensures that promises remain grounded in reality and are aligned with the current state of the system. This enables agents to easily adapt to changes as they arise.
Promise Theory: Emergent Coordination
In Promise Theory, the concept of complex behavior arises from the collaboration of multiple autonomous agents. Each agent in this system makes promises about its behavior, leading to the emergence of sophisticated outcomes. Similarly, in a task-centric system, the interplay between agents (both AI and human) generates innovative workflows and solutions. These emergent patterns emerge as agents cooperate and interact in the context of tasks. This type of emergent coordination heavily relies on the promises made by each agent regarding the specific tasks they’re willing to undertake. As a result, the system becomes highly flexible and scalable, with tasks dynamically distributed based on the system’s ever-changing state. Over time, this constant process of task negotiation enables the system to optimize workflows. This optimization occurs as the system learns and adapts from the behaviors and actions of its agents, continuously refining and improving the overall performance of the system.
Promise Theory: Trust-Based
The concept of trust is given significant importance in Promise Theory, particularly in distributed systems. Trust is established through the reliance on promises, which requires agents to have faith in each other’s ability to fulfill their commitments. This emphasis on trust is especially relevant in task-centric systems, where successful task completion is contingent upon the trustworthiness of other agents. To cultivate trust in the system, various mechanisms can be employed to monitor and validate task completion, ensuring the fulfillment of promises. These mechanisms may involve tracking and verifying task progress, as well as holding agents accountable for fulfilling their commitments. In the event of a broken promise, such as the failure to complete a task, the system can respond by triggering escalations or fallbacks. Possible actions may include reassigning the broken task to another agent or adjusting a workflow to accommodate failure.
Promise Theory: Handling Uncertainty
One of the primary advantages of Promise Theory is its effective management of uncertainty. Unlike rigid commands, promises are voluntary commitments that can be adjusted or withdrawn as necessary. This flexibility is particularly valuable in task-oriented systems, especially when operating in unpredictable environments where tasks may not always be straightforward. For instance, when an AI agent encounters unexpected challenges in completing a task, such as encountering a data error or an unusual customer behavior, it can renegotiate the task. This could involve escalating the task to a human agent or modifying the scope of the task itself. This aligns with the mechanisms of promise-breaking in Promise Theory, where an agent can revoke a promise under specific conditions. This ensures that the system remains resilient in the face of uncertainty or failure.
Speech Act: Action-Oriented
Speech Act Theory posits that communication encompasses not only the exchange of information but also the execution of actions through speech. This theory contends that language transcends its role in conveying facts and extends to encompassing promises, commands, and inquiries. Within the framework of the task-centric paradigm, where tasks assume paramount significance in human-machine interactions, Speech Act Theory furnishes a theoretical framework for comprehending the dynamics of these interactions. According to Speech Act Theory, when individuals speak or write, their words possess the capacity to perform actions. These actions can be categorized into distinct classes, including assertives (statements of fact), directives (commands or requests), commissives (promises or commitments), and declarations (status updates or decision announcements). In a task-oriented system, every interaction, whether involving a human and a machine or two AI agents, can be viewed as a speech act. Hence, agents engage in communication to facilitate the assignment, acceptance, monitoring, or completion of tasks.
Speech Act: Task as Frames
From a broader perspective, each task within this paradigm can be perceived as a type of speech act. To elaborate, assigning a task can be likened to a directive speech act, where one party instructs another to carry out a specific task. Conversely, accepting a task represents a commissive speech act, signifying the commitment to fulfill the assigned task. Providing a status update on a task corresponds to an assertive speech act, conveying information or updates about the task’s progress. Lastly, completing a task embodies a declarative speech act, indicating the accomplishment or fulfillment of the task. By conceptualizing tasks as speech acts, the system becomes more explicit and structured in terms of communication. This shift enables both human and AI agents to interact more effectively, as each agent can not only comprehend the shared information but also discern the underlying intent and further actions.
Speech Act: Intent & Interpretation
Speech Act Theory focuses on the purpose of communication. It goes beyond the literal meaning of a message and considers the intended action behind it. Applying this theory helps AI systems go beyond information processing and better comprehend the meaning behind communication in task-based systems. This is especially important for AI systems taking on agentic roles, where understanding user intent and coordinating actions between agents is vital for successful operation.
Speech Act: Performance & Performatives
Within Speech Act Theory, performatives are declarative statements that assume the capacity to execute upon utterance. When an AI agent makes a promise, the very act of making the promise constitutes a commitment to perform the specified action. This concept aligns with Promise Theory, which posits that agents engage in commitments to tasks. The notion of performativity underscores the inseparable link between communication and action. By integrating Speech Act Theory, every interaction within a task-oriented system transforms into either a task action or a promise to execute a specific task.
Speech Act: Task Negotiation
In multi-agent processes, such as customer journeys, negotiations are prevalent. These negotiations encompass various aspects, including task assignment, timing, method, and objectives. Speech Act Theory serves as a framework for these negotiations. For instance, when an individual assigns a task to an AI agent, it’s akin to issuing a command. The AI agent subsequently pledges to complete the task by a specified deadline. Should the task require modification, either the individual or the AI agent can modify the status or renegotiate the terms. This structured approach to task negotiation facilitates mutual understanding of roles and expectations, thereby reducing confusion and enhancing cooperation between humans and machines—an ideal synergy in the context of business process automation and Agentic AI.
Promise Theory emphasizes that promises are voluntary—agents autonomously decide whether to make a promise or not. This voluntary nature also resonates with Speech Act Theory, where agents aren’t merely responding to commands but are engaged in dialogue that reflects their autonomy. In Promise Theory, promises can be renegotiated or retracted under certain conditions. The renegotiation of a promise, in this case, is expressed through speech acts, where the agent updates or modifies its commitment based on new information or constraints. By incorporating speech acts into the renegotiation process, systems can manage dynamic changes in task management more fluidly, ensuring that promises remain realistic and achievable in light of evolving conditions.
Joint Action: Mutual Coordination
The integration of Joint Action into the task-centric paradigm introduces a pivotal element of mutual coordination and interdependence, complementing the existing frameworks of Promise Theory and Speech Act Theory. While Promise Theory and Speech Act Theory primarily concentrate on individual commitments and the formalization of intentions, Joint Action redirects the focus toward the collaborative endeavors of multiple agents in pursuit of a shared goal. Joint Action acknowledges that agents don’t operate in isolation but rather continuously adapt their actions in response to the behaviors and signals exhibited by other agents. This dynamic and interactive approach establishes a system where agents actively monitor and respond to one another, ensuring the seamless progression of the task at hand. While Promise Theory provides a formal structure for individual commitments, Joint Action underscores the socially interactive context in which these commitments are made, where agents rely on immediate feedback from each other. This emphasis on real-time interaction and feedback ensures that agents maintain alignment as the task evolves.
Joint Action: Shared Intentions
In Promise Theory, individual agents make promises based on their autonomous abilities to fulfill them. However, in complex task environments, especially those requiring human-AI collaboration, agents need to develop and maintain shared intentions to complete tasks effectively. Joint Action provides a framework for this shared intentionality, enabling agents to pursue tasks with a common understanding of the goal. It introduces the concepts of shared goals and collective task management, where all participating agents are aware of their roles within the broader context of the task. This concept strengthens the connection between individual promises and collective action by ensuring that each promise aligns with the overall structure of joint, task-oriented behavior.
Joint Action: Interdependence & Flexibility
Joint Action is based on the concept that actions are interconnected and dependent on each other, unlike an isolated system where individuals work independently. In Joint Action, the success of one person’s actions often hinges on the actions of others. This implies that while formal commitments are made, individuals must remain adaptable and prepared to adjust as the situation evolves. Joint Action emphasizes the significance of maintaining dynamic flexibility in executing tasks. For instance, if someone commits to completing a task but encounters unforeseen circumstances, such as new information or shifting priorities, the ability of everyone involved to respond and adapt becomes paramount. Joint Action ensures that this interdependence is seamlessly managed within the task framework.
Joint Action: Collective Accountability
Promise Theory introduces the concept of individual accountability through an agent’s promise to fulfill a specific task. On the other hand, Joint Action focuses on a more collective form of accountability, where the success of the overall task relies heavily on the coordination and support between different agents. In this case, responsibility is both distributed and shared among the agents involved. If one agent faces challenges, the other agents can step in or adapt their actions to ensure the completion of the collective task. Promise Theory partially addresses this by introducing Super Agents, which consist of collections of agents and their respective promises.
Conclusion
By placing task-centricity at the core of our systems and interactions, we unlock a world of possibilities:
Enhanced Focus and Productivity: Enhanced focus and productivity: Task-centric interfaces present only relevant information and actions, reducing cognitive load and empowering confident decision-making.
Seamless human-AI collaboration: As AI agents advance, task-centricity facilitates collaboration. Humans and machines coordinate efforts, leveraging strengths for shared goals.
Dynamic Adaptability: In a world of constant change, task-centric systems shine. They offer the flexibility to reassign, reprioritize, and restructure tasks in real-time, ensuring that our systems can keep pace with the evolving demands of business and society.
Intelligent Automation: Framing interactions as tasks enables more intelligent automation. AI agents learn from patterns, suggest optimizations, and even complete workflows, freeing humans for strategic thinking and creativity.
Empowered Decision Making: Task-centricity doesn’t just present data—it contextualizes information within actionable frameworks. This approach transforms passive data consumption into active, informed decision-making.
The journey to task-centricity is challenging, requiring a shift in system, interface, and AI agent design. However, the rewards are immense, opening the door to unprecedented productivity, innovation, and human-machine symbiosis.
Back to the Future
A good while back, I presented a slide about machines working with humans to create virtual worlds and explore past, present, and future memories using models that can control behaviors and outcomes. Recent advancements confirm that this idea is accurate, which is common for technology inspired by sci-fi stories.
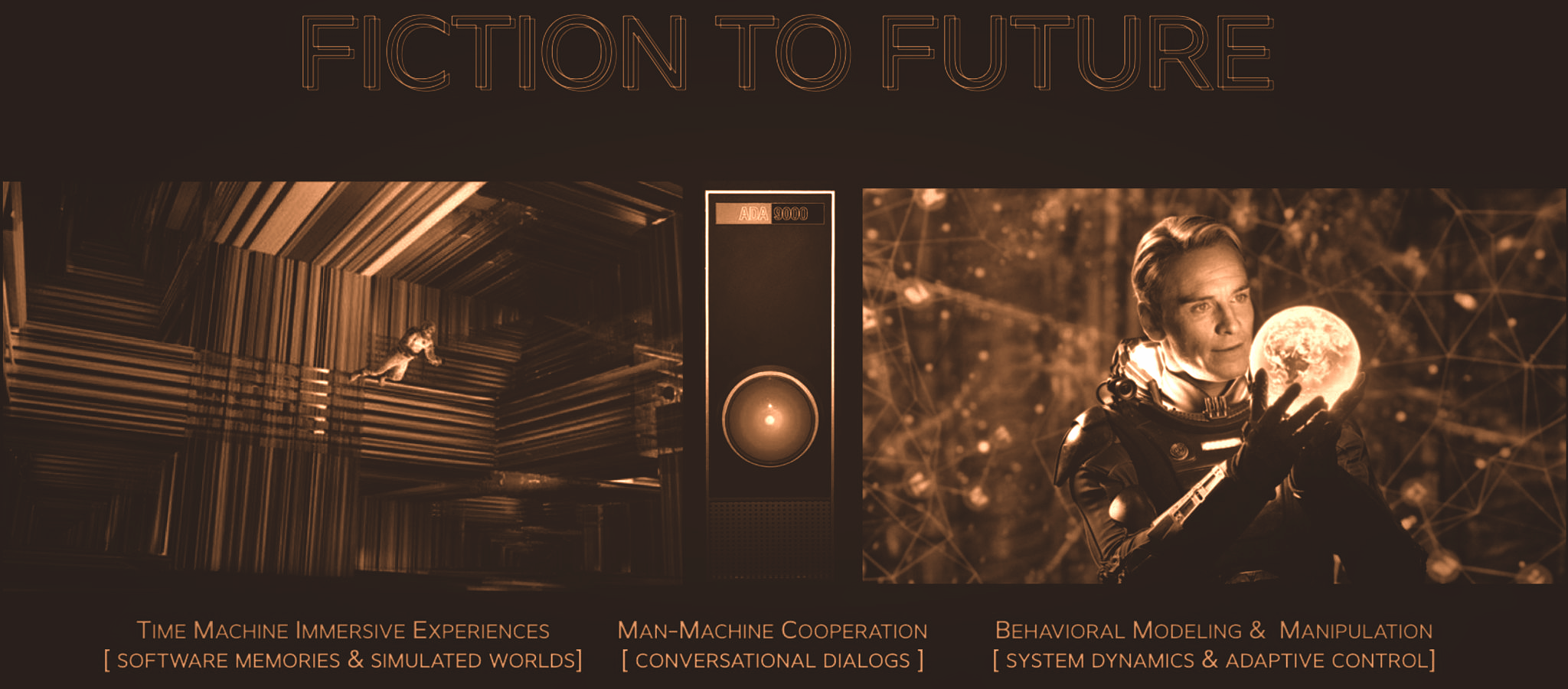