A Manifesto for Human-Centric Computational Systems
For decades, we’ve built computational systems under a grand illusion: that by mastering data collection, storage, and movement, intelligence and insight will naturally emerge. The reality we face today across observability, digital transformations, data engineering, and artificial intelligence proves otherwise. Our systems are efficient but not effective. They process but don’t understand. They inform but don’t enlighten. This isn’t just a technical failure—it’s philosophical. We’ve engineered complexity but not comprehension. We’ve built means for data but not for meaning.
The inherent subjectivity of meaning poses a significant challenge for computational encoding.
While meaning is inherently personal and contextual, it isn’t purely individual—it emerges from shared cultural frameworks, common cognitive patterns, and collective human experience. These shared structures provide a foundation for developing computational systems that can approximate human understanding.
Just as human societies develop common interpretive frameworks despite individual differences, we can design systems that recognize and work with these shared patterns of meaning-making.
The key lies not in attempting to create universal, objective meanings, but in building systems that acknowledge, mirror, and work with the intersubjective nature of human understanding.
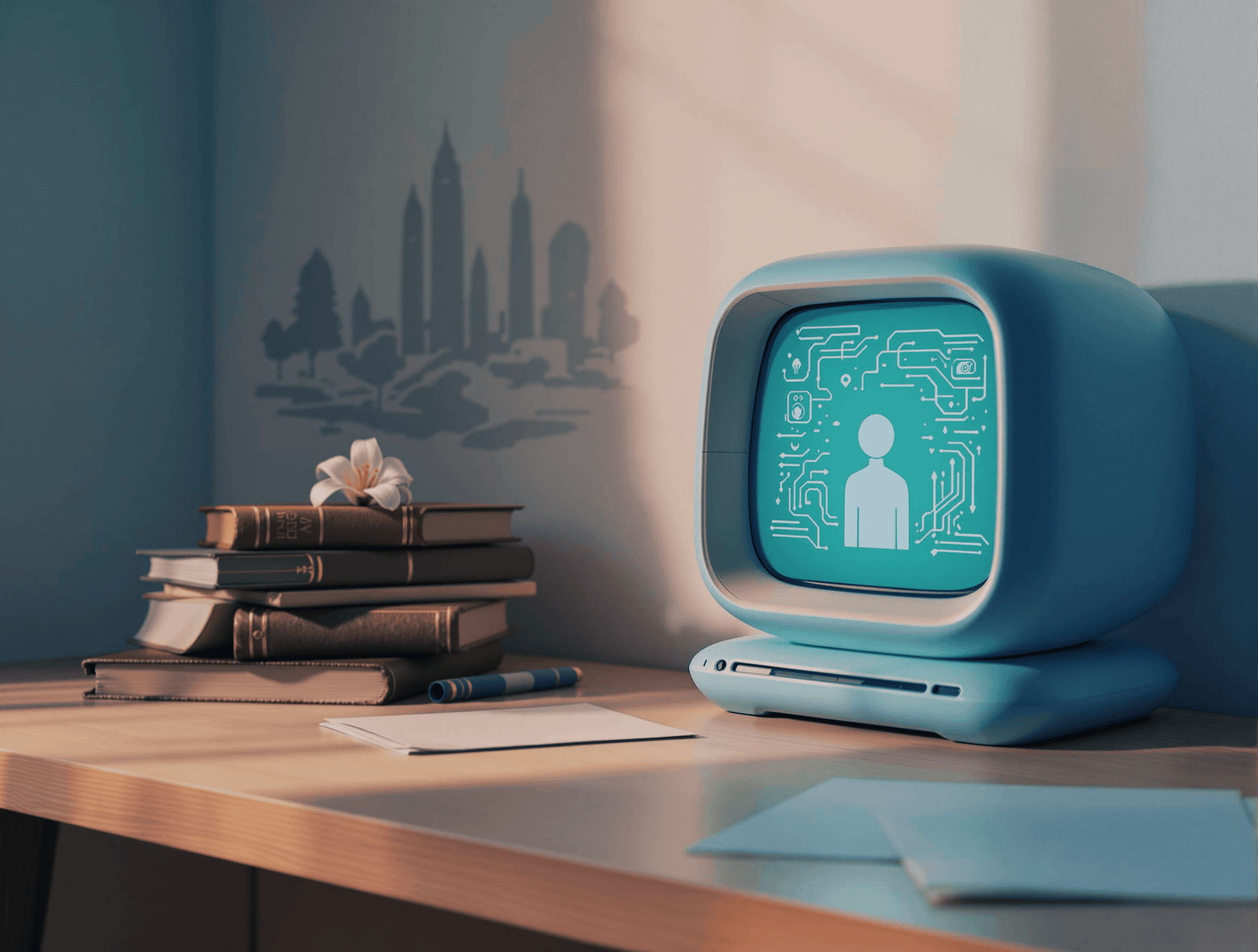
Through transparency and explainability, we can bridge the gap between computation and human interpretation. When systems make their reasoning processes visible and accessible, users can adapt and personalize their interpretations based on their contexts and needs. This fosters a dynamic relationship where meaning isn’t solely encoded by the system but co-created through interaction with users.
By acknowledging the role of cultural and cognitive frameworks in meaning-making, we can design systems that learn from and adapt to diverse interpretive communities, rather than imposing a singular model of understanding.
This approach recognizes that meaning exists not in isolated data points but in the rich network of relationships between information, context, and human understanding.
Our understanding of the world stems from our physical experiences. These embodied interactions profoundly influence how we perceive and interpret reality. Although perfectly replicating this embodiment computationally remains a significant hurdle, we can approximate it by integrating multimodal data processing and simulations.
The incorporation of sensory information, spatial reasoning, and simulated physical engagement enables the development of systems possessing a more robust and nuanced understanding of the world and its significance.
The Crisis of Meaning in Computational Systems
At the heart of every computational system lies an assumption that data is the foundation of intelligence.
This assumption is flawed. Data isn’t knowledge, nor is knowledge wisdom. The missing piece, the layer we’ve ignored, is semiotics—the study of how meaning is created, interpreted, and communicated.
Without this layer, our systems remain blind to the human reality they are meant to serve. Consider the following:
- Observability is drowning in noise because it tracks metrics but does not understand the concept of significance.
- Data engineering is obsessed with movement and structure but rarely questions whether the data itself holds relevance.
- Digital transformations focus on technology consolidation rather than making systems more interpretable and meaningful to users.
- Artificial intelligence recognizes patterns in language but does not grasp language as a vessel of human thought.
This isn’t a matter of interface design or usability. This is about the fundamental way we design systems—how they process, encode, and surface meaning. Without a semiotic foundation, all computational efforts amount to mere logistics: moving, transforming, and optimizing data with no deeper understanding of its significance.
The Three Aspects of System Intelligence
Every complex system where humans interact with computation depends on three core aspects:
- Observability – The ability to perceive system state.
- Controllability – The ability to influence system behavior.
- Operability – The ability to sustain and evolve the system effectively.
Today all three are broken because they can’t derive and represent meaning.
Observability provides raw data and telemetry but fails to distinguish between noise and meaningful patterns. Without semiotic structures, systems can’t elevate what’s important.
Controllability assumes mechanistic cause-effect relationships, yet human decision-making relies on context, narratives, and symbolic reasoning—none of which are captured computationally.
Operability is treated as a maintenance function rather than an adaptive system of meaning-making, making it impossible for organizations to truly steer their computational ecosystems over time.
The industry’s misinterpretation of cybernetics underscores our broader failure to comprehend the significance of meaning in computational systems. While we’ve enthusiastically embraced cybernetics’ technical components, such as data collection, sensory input, and feedback loops, we’ve overlooked its fundamental insight into the interconnected nature of intelligent systems. Cybernetic systems aren’t merely about gathering data or implementing control mechanisms; they’re about constructing meaningful feedback loops that facilitate comprehension, adaptation, and purposeful behavior. This selective application of cybernetic principles has resulted in systems that possess the capability to measure but can’t comprehend, react but fail to adapt, and collect but fail to grasp.
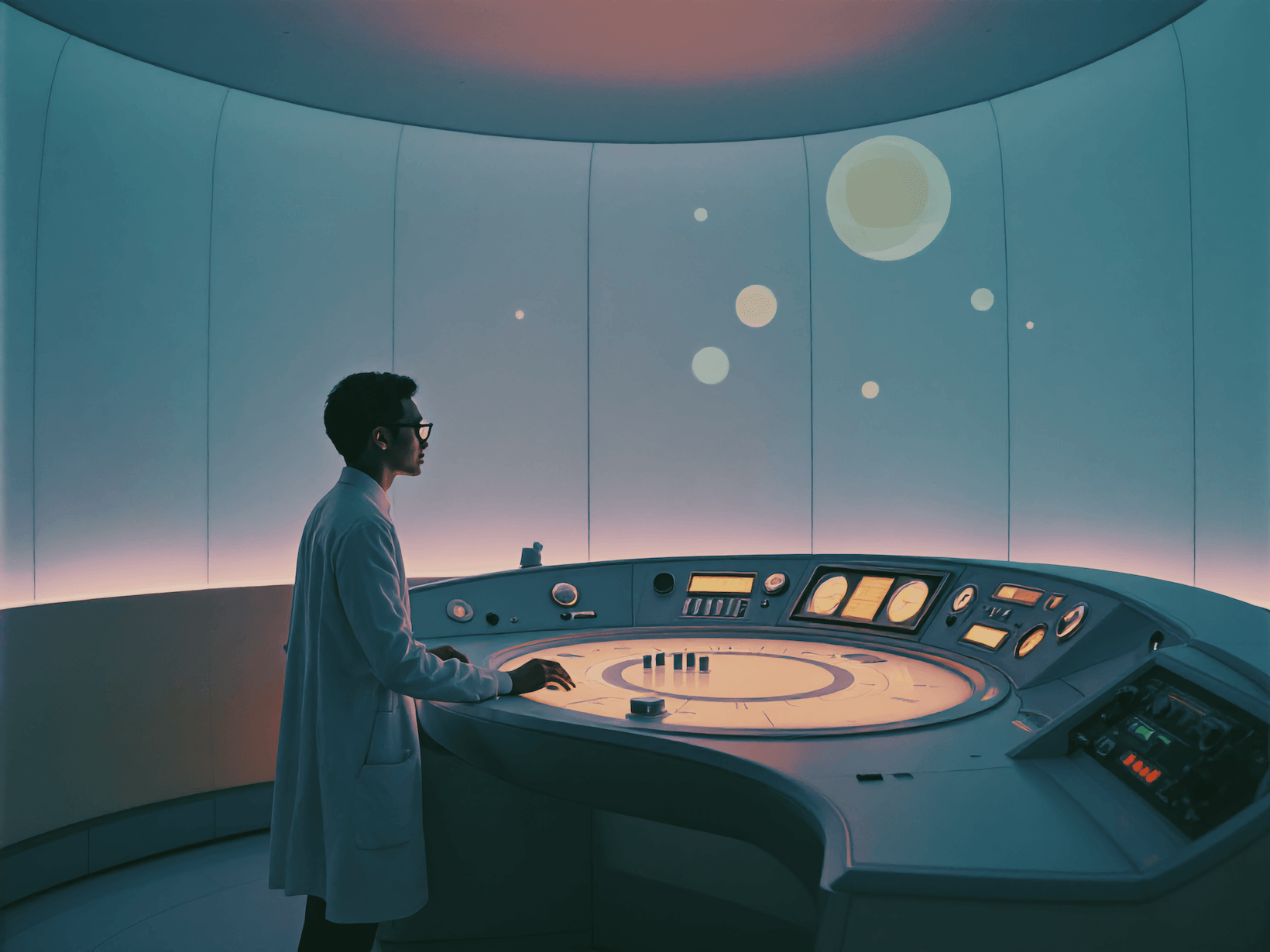
By revisiting cybernetics’ original vision of integrated, purpose-driven systems, we can commence the process of bridging the gap between mere computation and genuine intelligence. This entails perceiving observability, controllability, and operability not as distinct functions but as interconnected components of a singular, meaning-making system—one that possesses the capacity to learn, adapt, and evolve through meaningful interactions with its environment and the individuals it serves.
The limitations of AI today mirror those of all other software systems. It can process vast amounts of language but lacks semantics, context, and comprehension. It doesn’t “understand” language; it merely maps patterns to probabilities. What’s missing? The same thing is missing from our data lakes, dashboards, and digital twins: a model of meaning, a structured way of ensuring that outputs align with human cognition, interpretation, and action.
Engineering for Meaning
If we’re to move beyond the inefficiencies and limitations of today’s systems, we must reframe our approach.
Observability must move beyond collecting logs and metrics to contextualizing system states as signs. It must tell a story, not just emit data. Data pipelines shouldn’t just move information; they should surface why data matters.
We need systems that curate, contextualize, and refine—not just transport and store. A system shouldn’t just “run”—it should assist, interpret, and communicate in ways that align with human sense-making.
Operability should be seen as a cognitive function, not just a maintenance concern. Systems shouldn’t replace human intelligence but extend and enhance it by reflecting human ways of making sense of the world.
Prioritizing efficiency at the expense of meaning in computational systems is perilous, potentially fostering bias, disseminating misinformation, and eroding public trust.
The escalating reliance on such systems necessitates a shift towards prioritizing semantic understanding to ensure efficacy, ethical operation, and the mitigation of risks inherent in unchecked automation.
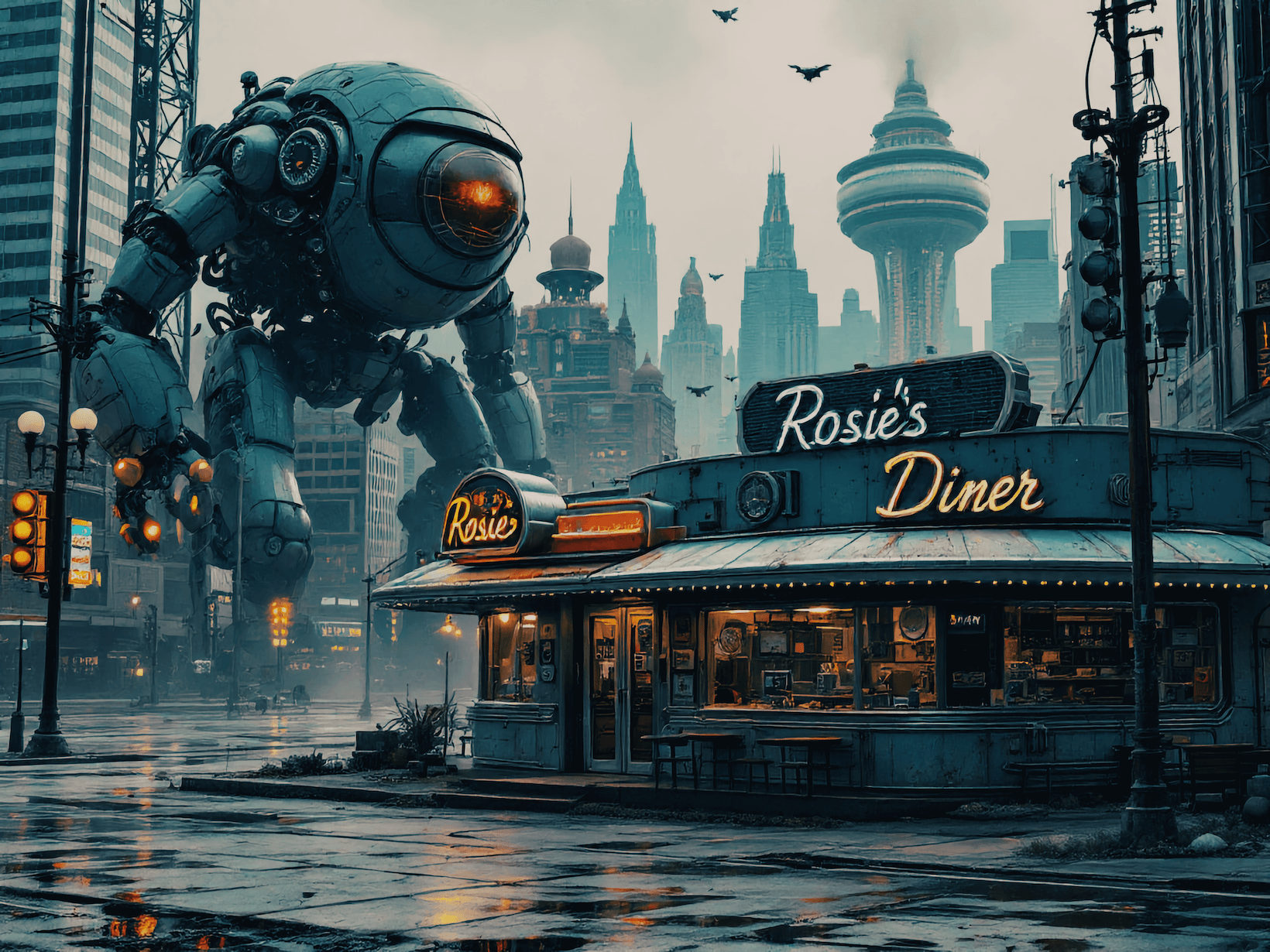
To bridge this gap, we must embrace semiotics—the study of signs and symbols and their use or interpretation.
Semiotics provides a framework for understanding how meaning is created and communicated. Specifically, by moving beyond the syntactical concerns (structure) onto the semantics (meaning), and pragmatics (context) of perception, we can build systems that move beyond mere pattern recognition to true comprehension.
The next frontier of AI isn’t just more data or larger models. It’s the integration of semiotics, cognitive science, and philosophy to build systems that not only predict patterns but also structure meaning.
A Call to Action
We must rethink our computational architectures from the ground up—not just in terms of efficiency, but in terms of their ability to support human sense-making and intelligence. This isn’t an incremental change. It’s a paradigm shift—from moving data to understanding meaning, from optimizing computations to augmenting cognition.
The future of computation isn’t in more powerful machines. It’s in more meaningful ones.
This paradigm shift profoundly impacts numerous fields, including observability, data engineering, digital twins, digital transformation, and artificial intelligence. Observability will transition from metric tracking to insightful interpretation for effective decision-making. Data engineering will prioritize data relevance and context, moving beyond mere storage to curated, insightful information. Digital transformations will focus on user-centric interpretability, transcending simple technological consolidation. Finally, AI will emulate human comprehension, integrating semiotics and cognitive science to create systems capable of nuanced understanding of language and thought.
To advance, we must redesign the fundamental elements of complex systems—observability, controllability, and operability—grounding them in inherent meaning. Observability needs to contextualize system status, providing meaningful narratives readily grasped by human intellect. Controllability should illuminate the actual effects of system adjustments, ensuring users comprehend the ramifications of their interventions. Operability should be viewed as a cognitive process, where systems facilitate, interpret, and communicate to bolster human understanding.
This integrated strategy guarantees technology acts as a genuine collaborator in human advancement, fostering innovation that’s both purposeful and ethical.
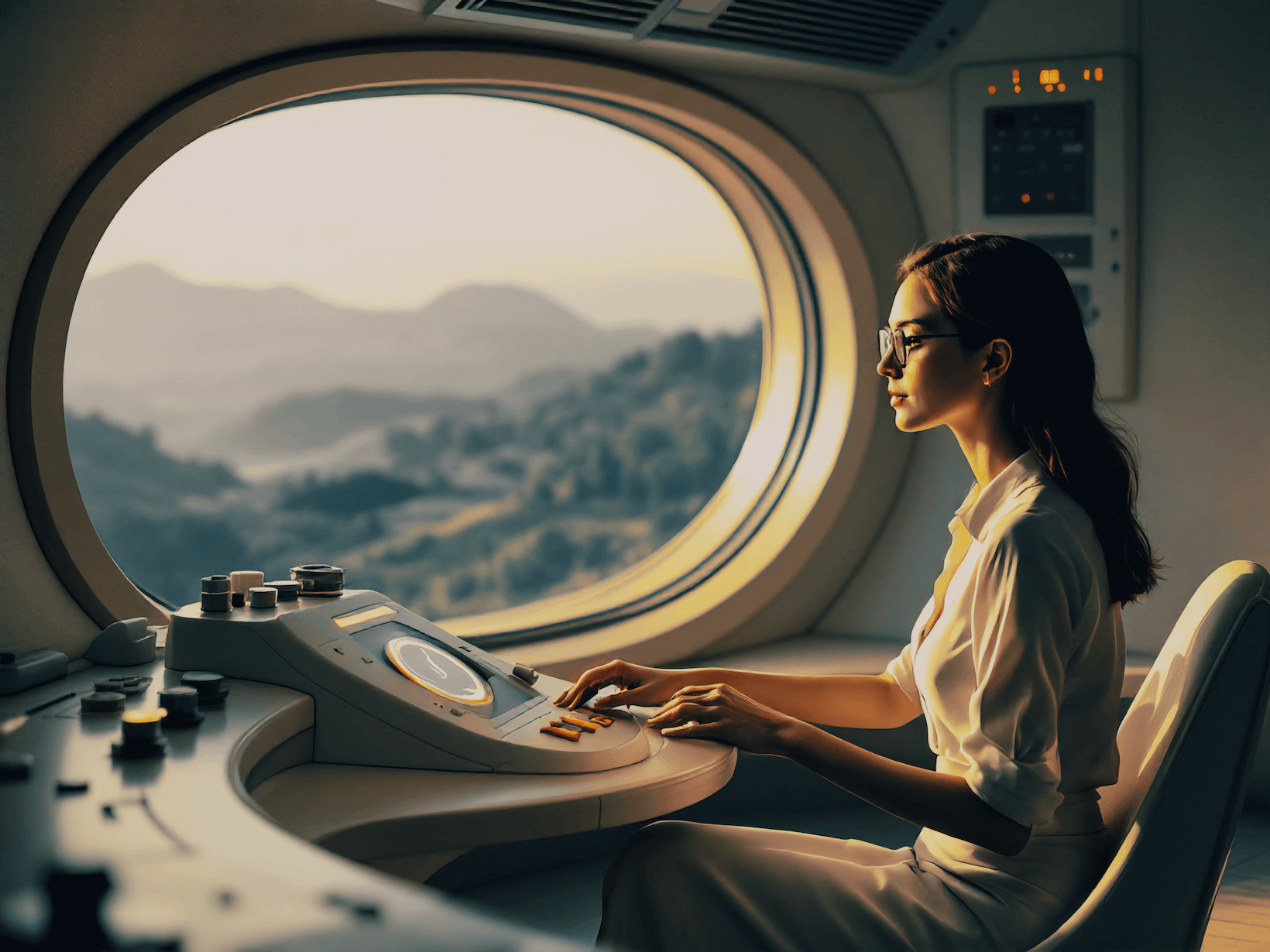
Realizing this vision necessitates interdisciplinary collaboration. Cognitive scientists, philosophers, and technologists must synergistically develop frameworks and methodologies integrating semiotics into computational architectures.
This collaborative endeavor will yield systems capable of not merely predicting patterns but also constructing meaning in alignment with human cognitive processes and interpretive frameworks.
The future of computation hinges on creating systems that emulate thought, not simply calculation, thereby cultivating a profound symbiosis between technology and human experience.
Ultimately, the manifesto’s call for meaning-centric systems is a call to reimagine our relationship with technology.
It’s about creating systems that augment our abilities, respect our cognition, and enhance our understanding of the world. This paradigm shift isn’t just about building better machines; it’s about building more meaningful ones—systems that serve human progress and drive innovation that’s both impactful and ethically sound.
By prioritizing meaning, we can forge a future where technology is a true extension of our intellect, fostering a more intuitive, responsive, and enriching interaction with the digital world.
Going Back to the Source
A fundamental reorientation of technology education is necessary to facilitate the transition to meaning-centric systems. This necessitates a move beyond technical expertise to encompass a comprehensive understanding of human cognition, semiotics, and ethical considerations. Curricula should prioritize interdisciplinary collaboration, rigorous critical analysis, and the design of systems that are both efficient and ethically responsible. Moreover, cultivating a societal appreciation for understanding as paramount, surpassing mere computational proficiency is crucial for the meaningful advancement of computing.
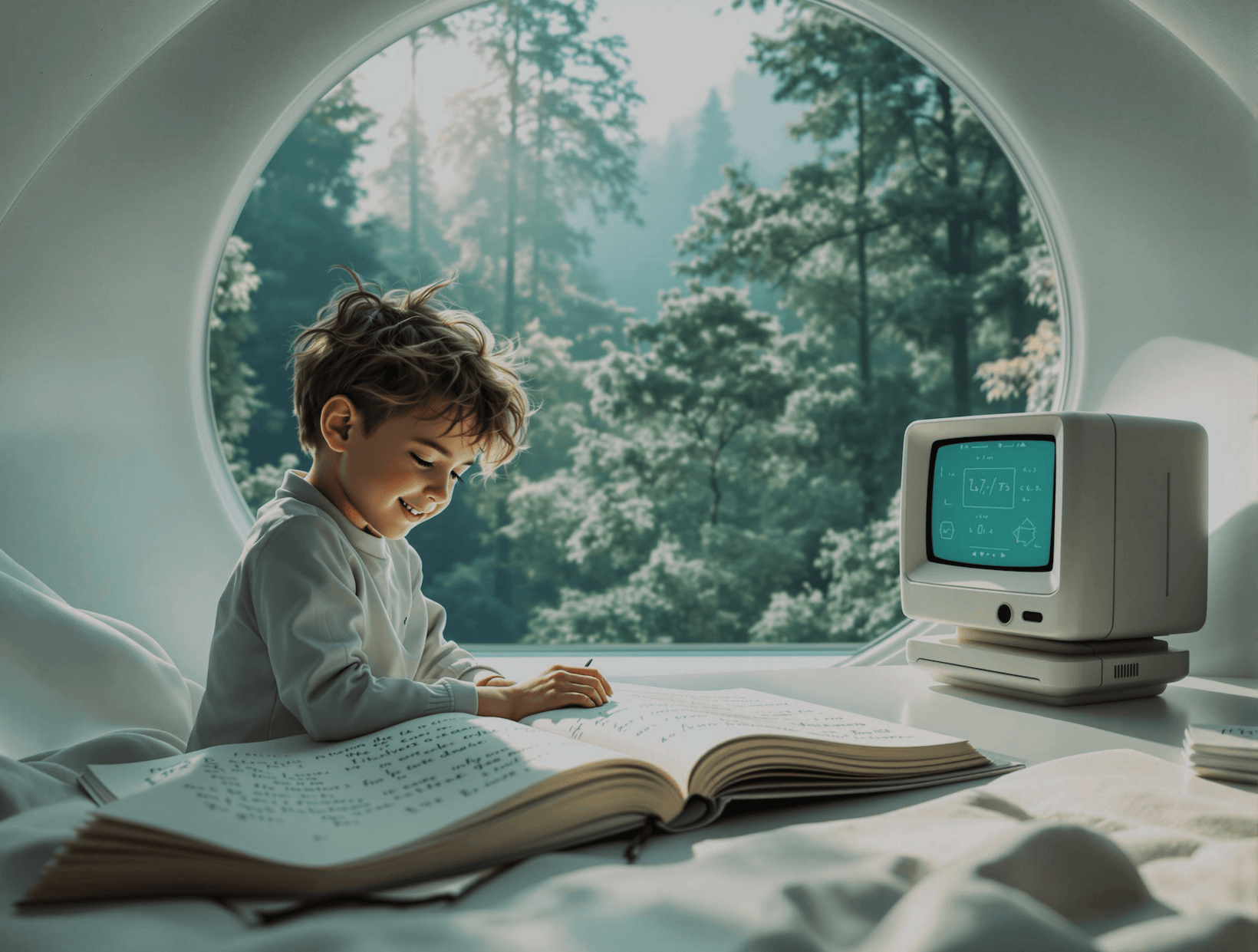