Observability Purpose
Observability is purposefully seeing a system in terms of operations and outcomes. In control theory, this is sometimes simplified to monitoring inputs and outputs, with the comparative prediction of the output from input, possibly factoring in history.
Observability is about more than just collecting data. The data is a means for something far more critical – the inference of stability (or predictability) in the reliable operation of a system. Is the system serving the needs of its consumers (users)?
A key objective of operational intelligence, whether artificial or not, is to accurately assess whether the system has and is currently operating reliably and to determine whether an intervention in the form of change (actuation, configuration) is required when the assessment is not favorable, or there is a prediction that things (situation) are about to change adversely. HAL 9000!
Situation Awareness
Consider the following setting. Alice and Bob are having a meeting in which they ask and answer questions of and from each other. Each party in the meeting can be seen as a system. They can also be seen as playing the role of service in the conversation.
When Alice asks Bob a question, Alice is a Consumer of Service named Bob. When Bob answers Alice, he is a Service Provider to a Consumer named Alice, specifically, a Producer of answers to questions from Alice. There are three possible systems, boundaries if you will, an observer can demarcate. The first two are the participants. The third is the meeting, or the process of meeting, temporarily enclosing the other two systems. We can view it as a proxy for the organizational entity that both parties belong to.
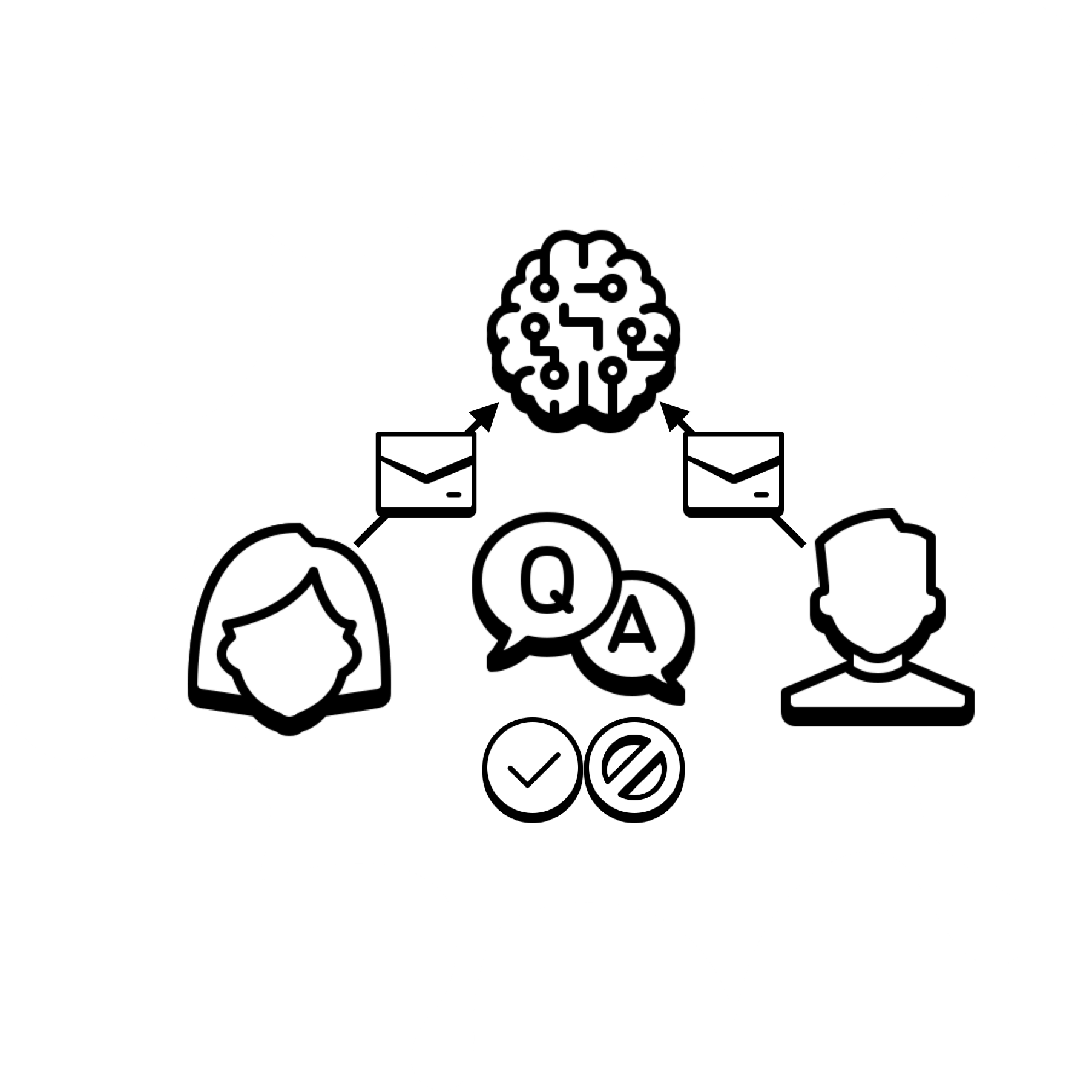
Observer Signals
Now let’s bring observability into the picture by introducing an Observer. The Observer need not be present in the meeting but instead can rely on each party to emit signals (operation, outcomes) and the context, including source and subject.
When Alice asks Bob a question, the Observer will receive an event with the source being Alice, the subject being Bob, and the signal being ASK. When Alice receives an answer from Bob, the Observer will receive an event with the source again being Alice, the subject being Bob, and the signal being ANSWER. When Bob asks Alice a question, the roles reverse, he becomes the source, and she becomes the subject. A future post will introduce an orientation aspect of the emittance of signals. Let’s keep it simple for now.
Assessment Status
The primary purpose of the Observer is to assess the effectiveness of the meeting and the reliability of each of the parties acting as service providers. This inference process can be complicated if the meeting is online, where the communication can be temporarily disconnected. Bob could reply to a question asked by Alice but which she never received. Multiple truths are possible.
In the more traditional approach to IT operations management, the truth comes from one source, the service. With observability, there is a shift to the consumer (caller). But each has only a partial picture of the situation. We can ask Alice how she felt she performed when she was questioned. We can also ask Alice how she thinks Bob performed when she asked him a question. Each system, service, or party can report on its performance as well as the performance of the other party. Both parties can also report on the effectiveness of the meeting. Today, observability tooling picks one perspective and ignores all others. Simplistic, not simple.
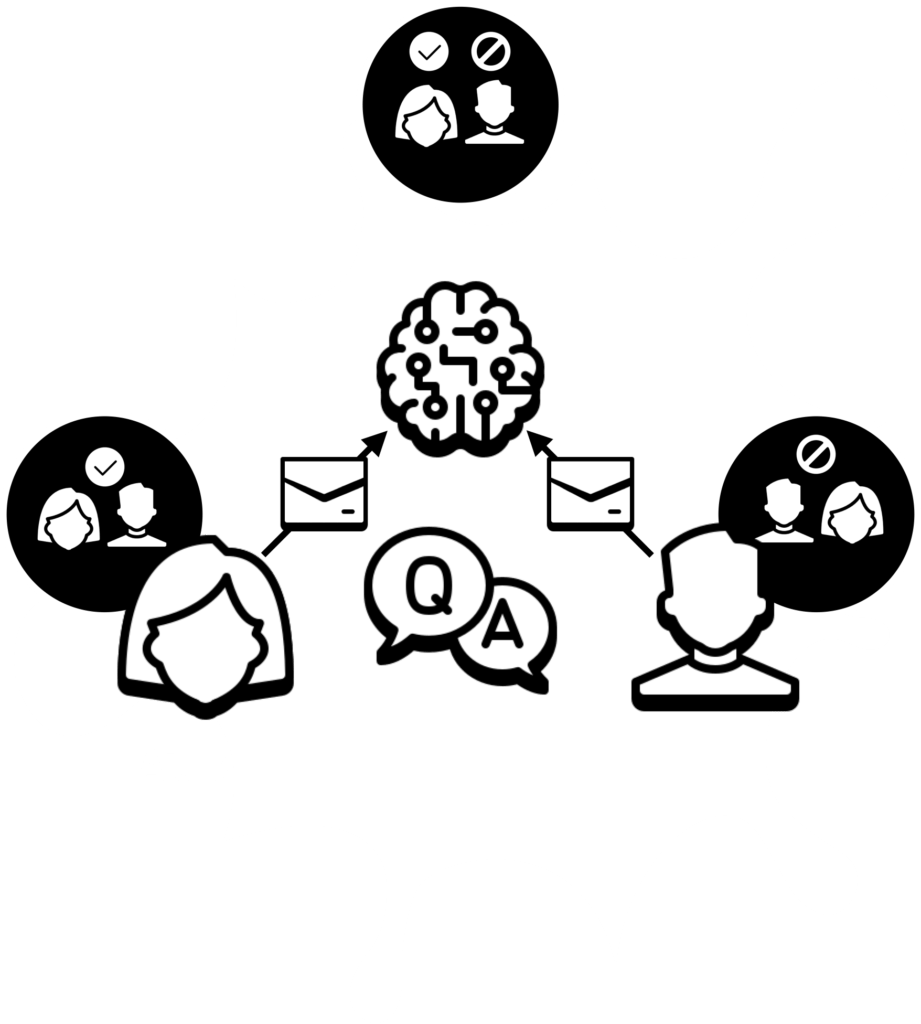
If we are to introduce intelligence, the Observer must also assess the status of a process at various scopes and the sensitivity to specific signals or sequences of signals that might not necessarily be seen as of equal importance in a context. The intelligence must reconcile the possible difference in opinion that each system can (in)form, which we have named service cognition.
Something to note is that the Observer is not concerned with the content quality of the questions and answers. Many site reliability engineering teams forget this and get bogged down collecting content data, which can be helpful for the user and application-level diagnostics but is far too noisy for effective and efficient service-level monitoring and management.