This article was originally posted in 2020 on the OpenSignals website, which is now defunct.
Course Change
Today, companies face mounting pressure to demonstrate speed and agility in an ever-changing and increasingly competitive environment. Information technology is seen as a critical enabler in adjusting to market shifts and threats and increasing customer expectations while evolving and improving offered services. For an organization to improve its capability to change, the underlying systems supporting it must also change, generally at a much faster rate, to coherently connect the past, present, and predicted future with some degree of continuity.
Breaking Down Change
While the business focuses on charting a course from one change point to another on a timeline of services and market evolution, the computing infrastructure and hapless engineering teams must deal with the only thing worst than change itself, and that is the transition period each of these points, moving from a discrete view of the world to one that is continuous and complicated. Not to worry, engineers believe they have everything figured out – make more minor changes but faster, much like a pilot in a fighter plane using the OODA loop to get within the enemy’s loop. The opponent is business, and engineering proactively maneuvers and anticipates re-orientations. That is until the question is raised – how to observe and orient with an ever-growing big pile of low-level data hooked up to a bunch of dashboards.
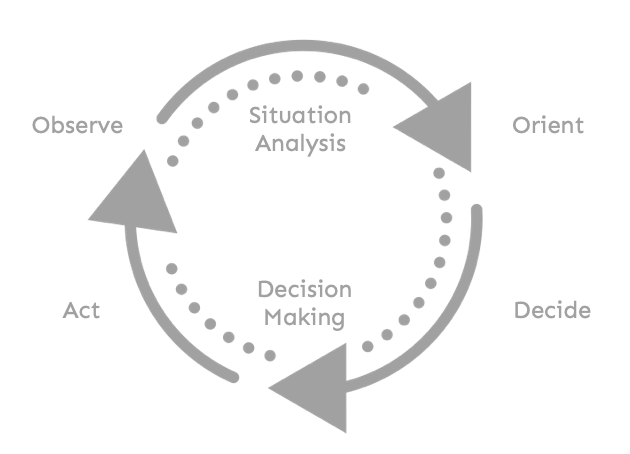
Situation Analysis: Observe • Orient
The OODA loop emphasizes two critical environmental factors – time constraints and information uncertainty. The time factor is addressed by executing through the loop as fast as possible. Information uncertainty is tackled by acting accurately. The model’s typical presentation is popular because it closes the loop between sensing (observe and orient) and acting (decide and act). The Observe phase focuses on data acquisition and information synthesis about the environment, unfolding situations, and interactions. The goal of the Orient phase, which follows the Observe phase, is to make sense of collected observations from an operational viewpoint.
Decision Making: Decide • Act
Understanding the situation and potential scenarios that may follow from this point in time depends on the expertise and experience of observers – situation assessors and decision-makers. The next step in the process is the Decide phase, in which information fed from the Orient phase determines the appropriate action(s). Finally, the Act phase is where the course of action decided upon earlier is implemented. The cycle repeats with further observations.
The Limits of the Loop
However, there are problems with the OODA model. It does not detail how later phases steer and influence, specifically, self-regulate, earlier phases, and vice versa. Invariably, it is seen and described as sequential without the ability to exit prematurely and re-enter. It also omits attention, memory, and the cognitive representation of world states and models. It also lacks any deliberate planning and learning phases. The OODA model is broad in its description of the decision-making process. Other than listing some of the factors pertinent to the Orient phase, it offers very little on implementing it.
Big Data Addiction
The OODA model’s most significant issue is that it does not capture the encompassing goal and objectives, making the loop reactive rather than proactive. The model appeals to one of the worst trends within software engineering and services operations – big data addiction. Here effective operations management and its decision-making are seen as merely a problem of insufficient data collection and information construction. Unfortunately, expanding the capacity to transmit more and more data to the cloud has not improved situation awareness; in fact, it seems to have made it more difficult, if not impossible. It’s not just simple; it’s simplistic.
Drowning Firehoses
OODA and Big Data do not reflect how human perception and cognition work to direct attention and interpret sensor signals. OODA, and much of the work currently ongoing in the Observability space, incorrectly assume engineering is principally passively reacting to environmental-sourced events – this is never more so exemplified in the design of data-ladened monitoring dashboards fed by dumb data pipelines.
Goal Lead, Situation Driven
Successful service operations and management in dynamic environments enclosing highly complex systems depend on focusing on clear goals at various levels of composition and planning how to achieve and maintain them. OODA and Big Data approach human-and-machine cognition with a simplistic, mechanical, and data-centric viewpoint, utterly ignorant of situations and scenes, intentions and inferences, signals and sequences, services, and states – devoid of patterns and models that could help to more effectively direct both humans and computer attention in assessing current conditions, predicting future events, and tracking the results of scripted and curated system interventions. Data, data everywhere, and not a situation to be recognized.
Lesson: Cognition is Situated
Let’s stop for a moment and ask ourselves the question. How does one even imagine that a site reliability engineer (SRE) can go from hundreds, if not thousands, of distinct trace paths, metrics, and log patterns to formulate the current situation against which it is compared with past prototypical patterned situations? And if you are naive enough to believe that machine learning will solve this issue, then ask yourself how one, human or machine, can honestly and with some degree of certainty predict from such contextless data the transitions between situations and explain this to an engineer in a communicable form. Cognition is situated, yet the industry keeps offering solutions with even more significant problems that disconnect us from the situation beyond the fact that we’re slowly sinking.