This article was originally posted in 2020 on the OpenSignals website, which is now defunct.
The OODA Loop
In a previous post, we called out several issues with the standard or typical example of the OODA loop. One particular point was the lack of detail surrounding the Decide phase of the model. While OODA explores some of the factors involved in the Orient phase that feeds into the Decide phase, it offers minimal elaboration on what is relayed and how it might be reasoned about. Here, we can augment the OODA loop with another decision-making model, the Recognition-Primed Decision (RPD) model of rapid decision-making.
Recognition-Primed Decision Model
The model asserts that individuals assess the situation, generate a plausible course of action (CoA), and then evaluate it using mental simulation. The authors claim that decision-making is primed by recognizing the situation and not entirely determined by recognition.
The model contradicts the common thinking that individuals employ an analytical model in complex time-critical operational contexts. Multiple options are carefully evaluated, weighed, and compared before choosing a response or action. The analytical model works best with inexperienced individuals, whereas experts employ a more naturalistic decision-making method that is heuristic, holistic, or intuitive.
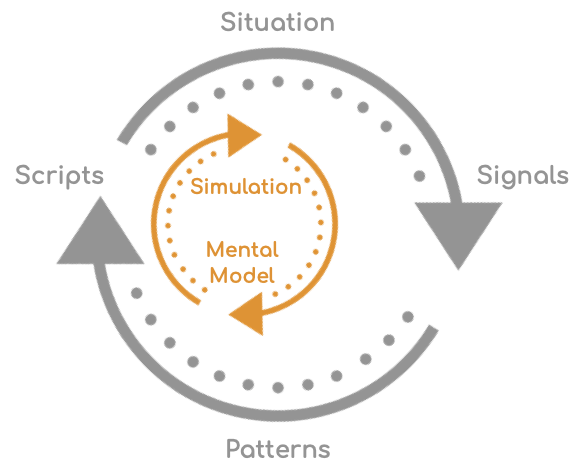
Situation Recognition
In the RPD model, an expert understanding of a situation depends mainly on the goals, cues, expectations, and typical actions within such situations (prototypical patterns). The RPD model has three components: matching, diagnosis, and simulation. The matching component attempts to identify the current situation from the memory of prototypical situations. Further diagnostics are obtained if the situation is not recognized and (online) learning is engaged. Here pattern recognition is central to decision-making. A pattern consists of cues, spatial and temporal relationships, and cause-and-effect chains and reflects the operational goals and expectations.
Charting a Course
The intelligence or expertise we commonly hear in mission-critical situations is the extensive knowledge of patterns that make it extremely easy to identify the small but critical states a system is within or about to enter. Once a decision, or choice of action script, is made, a mental simulation of the anticipated consequence is done, and the expected outcome is compared with the goals. Here an effective and efficient mental model of the situation is paramount for a fast and accurate assessment. If the outcome is favorable, action is taken; otherwise, alternative scripts are evaluated. If none of the mapped scripts are acceptable, further diagnosis is initiated.
Reframing Observability
Most of us can readily recognize the essential aspects of this model in everyday life. Still, it is hard to pinpoint where our current Observability tooling approach supports complex distributed computing systems. The site reliability engineering (SRE) community currently emphasizes data collection, which is far too quickly and naively relabeled as information or knowledge.
Acquiring information on a system equates to projecting the system’s future states with much less uncertainty. How does a distributed trace, log, or event even come close to addressing such prediction capabilities and capacities? The lens (or model) from which we view the computing world has many engineers staring down at data and details, seeing trees, more so roots, while being utterly oblivious to the forest, ecosystem, and nature at play (the dynamics of action). We’re failing at first base.